Analyzing Key Competitors of Domino Data Lab
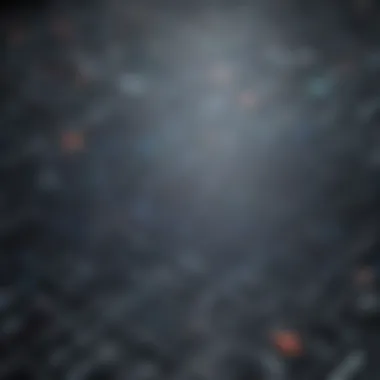
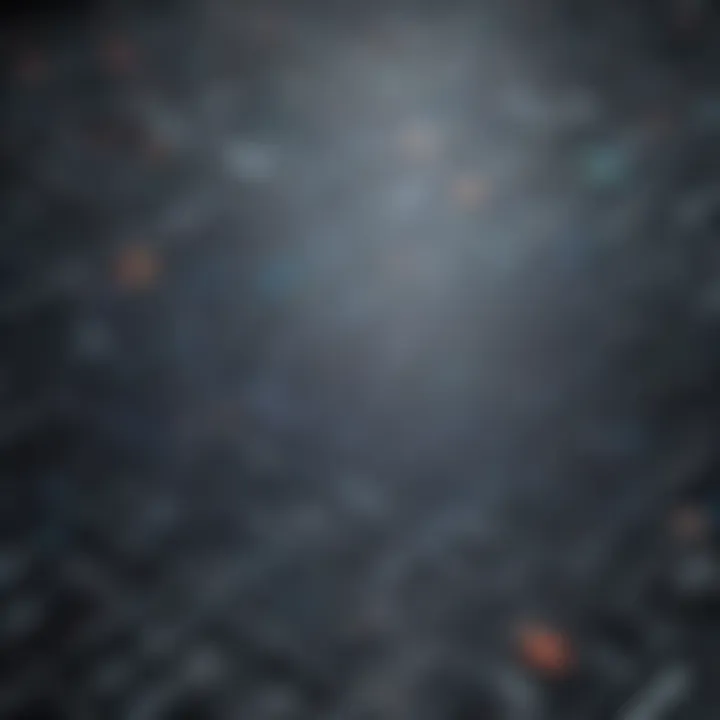
Intro
In today's fast-paced data-driven world, choosing the right data science platform can feel like navigating through a maelstrom of options. With businesses continuously chasing innovation, it becomes crucial to not only recognize the offerings of prominent players like Domino Data Lab but also to delve into their competitors. This exploration isn't just about awareness; it's about finding the tools that fit an organization’s unique needs.
The competitive landscape of data science and machine learning is becoming crowded, with various platforms emerging to capture a slice of the market. These alternatives often come packed with their own unique features, pricing structures, and target audiences.
Let’s embark on a comprehensive analysis, focusing on the strengths and weaknesses of key competitors, while exploring their functionalities, usability, and value propositions. By doing so, decision-makers can sharpen their insight into the best options available for their data operations and machine learning workflows.
Key Features of the Software
When assessing software platforms, particularly in the realm of data science and machine learning, understanding the key features is essential. This section aims to highlight the core functionalities and unique selling points that differentiate each competitor from Domino Data Lab.
Overview of Core Functionalities
- Collaboration Tools: Many platforms emphasize teamwork in data projects. For instance, IBM Watson Studio allows multiple users to work on the same project seamlessly, providing version control and feedback loops which is essential for larger teams.
- Automated Machine Learning: Companies like RapidMiner are pushing the envelope with automation, making machine learning accessible even to those who may not have extensive coding backgrounds.
- Integration Capabilities: Integration with various data sources and software stacks is another critical aspect. Tools like Databricks have garnered attention for their strong compatibility with cloud services like AWS and Azure, enabling seamless data import and processing.
Unique Selling Points
- User Experience: Platforms such as Alteryx focus heavily on providing an intuitive interface, simplifying the analytics process for non-technical users. A focus on usability can be a deciding factor for smaller businesses who may not have dedicated data teams.
- Customizability: Various competitors allow for personalized workflows and dashboard arrangements, catering to specific needs and preferences. This flexibility is particularly appealing for organizations that require a tailored approach.
"The right tool can transform a scattered process into a coherent operation, making it worth the time to analyze the available options."
In-Depth Software Analysis
An in-depth look at a software's strengths and weaknesses can provide pivotal insights for potential users. This section discusses the pros and cons of the prominent competitors, alongside measurable performance metrics.
Pros and Cons
- Pros:
- Cons:
- Speed: Many platforms are optimized for rapid data processing which is crucial in today's fast-paced business environments. For example, Google Cloud AI has demonstrated superior processing capabilities in handling large datasets.
- Support Resources: A wealth of learning resources can ease the adoption process. Platforms with extensive documentation and community forums, like Kaggle, can provide support when users encounter challenges.
- Cost Considerations: Pricing models vary significantly. Some tools, while feature-rich, can be prohibitively expensive for small businesses, which may limit their accessibility.
- Complexity: As platforms grow in capabilities, they can become overwhelming. Tools with steep learning curves may dissuade teams that require swift onboarding.
Performance Metrics
To objectively assess software platforms, one can consider several performance metrics:
- Processing Time: Evaluating how long it takes for a platform to carry out tasks can be indicative of its efficiency.
- Scalability: In a business environment where data volume can grow rapidly, how well does the platform accommodate increased workload?
- User Satisfaction: Feedback from active users can shed light on the day-to-day experiences with the software.
Understanding these facets aids in building a holistic view of the available platforms and empowers decision-makers to make informed choices that best meet their organization’s needs.
Foreword to Domino Data Lab
Understanding Domino Data Lab is vital to grasping the competitive data science platform landscape. This section serves as a springboard, launching into the realm of data science tools and their functionalities. Recognizing the purpose and strength of Domino allows professionals to better evaluate its alternatives. In time, they'll also appreciate the nuances that define successful implementations in various organizations.
What is Domino Data Lab?
Domino Data Lab is essentially a powerful working environment tailored for data scientists. Think of it as a sophisticated workspace where teams can collaborate on machine learning projects without stepping on each other's toes. Users can share code, reproduce results, and manage various environments, all in one place. Companies often regard it as the bridge between data teams and engineering. Just like a well-stocked toolbox, Domino equips data scientists with the necessary resources to tackle intricate problems.
The platform emphasizes reproducibility. Anyone who's dabbled in data knows the importance of running consistent experiments. Domino shines here, giving users the ability to track changes and ensure that results remain valid across different versions. Moreover, its integration capabilities can connect with various data storage tools or cloud providers, enhancing its adaptability.
Significance in Data Science
In today's data-driven world, the significance of Domino Data Lab cannot be overstated. It's not just another tool among many; it's a cornerstone for organizations striving to harness the potential of their data. It allows businesses to streamline their analytics, which can directly impact their bottom line.
By making collaboration seamless, Domino encourages teams to innovate and improves productivity. Think of it in terms of a relay race, where every team member must pass the baton smoothly for success. When data scientists can easily communicate and share insights, the entire organization benefits.
In an era where data is being generated at an unprecedented rate, the ability to analyze and derive actionable insights is crucial. Domino acts as a facilitator in this process, helping businesses make informed decisions. It's not just about having data anymore; it’s about making that data work for you.
"Data itself is not valuable, but the ability to analyze and make decisions based on it is what truly drives organizations forward."
Understanding these points ultimately leads to making informed choices regarding competitors. By appreciating Domino Data Lab’s role in data science, one can better analyze the market and weigh options against the landscape of available platforms.
The Data Science Market Landscape
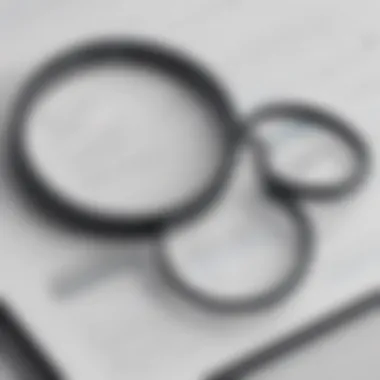
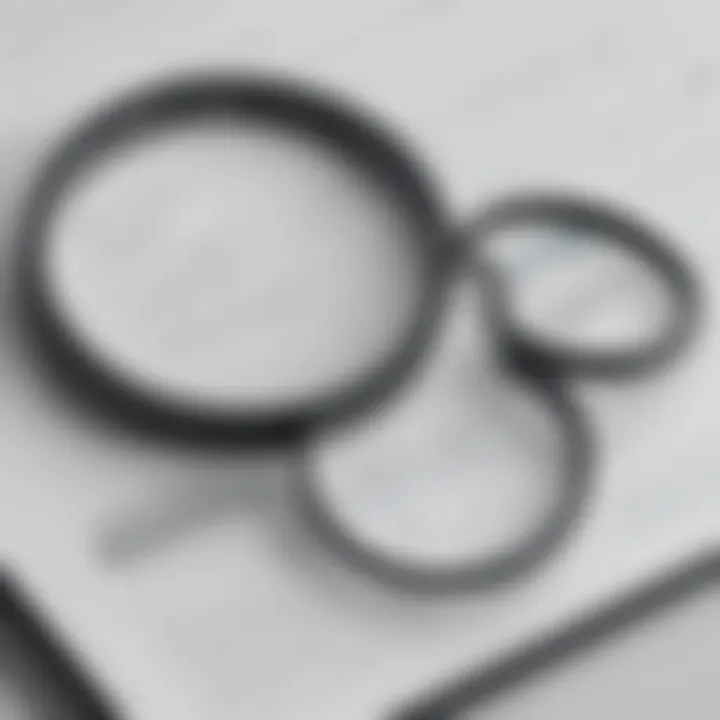
Understanding the data science market landscape is crucial for any organization looking to thrive in an increasingly data-driven world. This arena isn't just about crunching numbers; it's where innovation meets practicality, shaping the way we analyze, visualize, and implement data strategies. For businesses, especially small to medium-sized ones, grasping this landscape is vital for decision-making, resource allocation, and staying ahead of the competition.
Organizations are now faced with an overwhelming variety of tools and platforms tailored for data science. Therefore, knowing the strengths and weaknesses of these options becomes increasingly significant. In this article, we will dissect the current state of the data science market, elucidating the trends and technologies that hold sway over this evolving field.
Growth and Trends
The growth of the data science market hasn’t just been a blip on the radar; it's a full-blown phenomenon. Over the last few years, the proliferation of digital data has fired up the engines of analytics and machine learning. Businesses are increasingly recognizing the value of data as a key asset. According to industry reports, the global data analytics market is expected to reach over $300 billion by the end of this decade.
A few trends stand out in the current market:
- Increased Investment: Companies are pouring resources into data science initiatives. This funding isn't solely for hiring data scientists, but also for acquiring state-of-the-art tools and technologies.
- Emphasis on Automation: There’s a significant shift towards automating data processes. Automation not only cuts time but also reduces human error, making it a desired feature among data science platforms.
- Cloud Adoption: Many organizations are migrating their data workflows to the cloud. Cloud solutions provide scalability and flexibility while minimizing infrastructure costs, substantially benefiting smaller businesses.
Emerging trends indicate that as more businesses harness data, the demand for skilled professionals will continue to rise, leading to the expansion of educational programs in data science.
Emerging Technologies
The data science corner of the tech world is also getting a facelift with the introduction of novel technologies. Staying informed about these advancements is essential for making strategic choices. Some key technologies gaining traction include:
- Machine Learning and AI: These technologies are the backbone of data science. They enable predictive analytics and help synthesize insights from massive datasets.
- Natural Language Processing (NLP): With the increasing volume of unstructured data, NLP tools are becoming indispensable for interpreting human language, offering businesses the power to analyze customer feedback efficiently.
- Big Data Technologies: Solutions like Hadoop and Apache Spark allow for processing large datasets at unprecedented speeds, enhancing an organization’s ability to draw insights from their data.
- Augmented Analytics: This involves the integration of AI within analytics tools, allowing users—regardless of their technical background—to glean insights without needing sophisticated skills.
As these technologies continue to evolve, they alter not only how businesses approach data science but also how they compete with one another.
"In a world where data is king, understanding the landscape is like having the map to a treasure chest. Without it, businesses risk navigating blindly through challenges and opportunities."
In summary, the data science market landscape is not a static entity; it transforms and expands continually. Recognizing growth patterns and the significance of emerging technologies will pave the way for informed decision-making in the data science sphere.
Direct Competitors of Domino Data Lab
Understanding the direct competitors of Domino Data Lab is crucial for small to medium-sized businesses, entrepreneurs, and IT professionals. As the data science landscape expands rapidly, recognizing alternatives can aid in making more informed decisions. These competitors offer distinct functionalities and cater to various needs, from enhancing model deployment to providing advanced analytics. By taking a closer look at these rival platforms, organizations can discern which solution aligns most closely with their specific data operations and machine learning workflows.
DataRobot
Overview
DataRobot has carved a niche for itself in the automated machine learning space. It's designed for organizations that seek to streamline their predictive modeling capabilities without the need for extensive programming knowledge. DataRobot's ability to quickly produce robust models makes it a go-to platform for many. Its key characteristic is the automated approach to machine learning, allowing users to generate accurate models efficiently. However, this reliance on automation might be seen as a double-edged sword. Some users miss the flexibility of manual modeling, which is advantageous when dealing with complex datasets that require nuanced interpretation.
Key Features
Among DataRobot's standout features, its visual interface receives considerable acclaim. This user-friendly design enhances the accessibility of machine learning for non-technical users. A particular highlight is its extensive integration with various data sources and tools. This ability to connect seamlessly with existing data workflows is a significant benefit for organizations looking to enhance their data strategies. On the flip side, while the ease of use is praiseworthy, the depth of customization can feel limited for advanced users needing detailed control over their models.
Comparative Analysis
In comparing DataRobot with other platforms, its emphasis on automation stands out. This characteristic positions it as a favorable choice for organizations aiming for speed. However, when pitted against alternatives like O.ai, which offers more manual control options, DataRobot’s automated approach could be perceived as less flexible. The choice largely depends on the specific needs of the organization — the balance between speed and customization is a critical consideration.
O.ai
Product Overview
O.ai is known for its focus on open-source, scalable machine learning solutions. This platform caters to both technical and less technical users by providing a spectrum of functionalities. Its key characteristic lies in its strong community support and contributions, often leading to rapid enhancements in features. This open-source nature makes O.ai particularly attractive for budget-conscious businesses looking to leverage powerful machine learning tools without hefty licensing fees. The downside might be the steeper learning curve for those unfamiliar with coding or statistical methods.
Strengths
A considerable strength of O.ai is its performance in handling large datasets. Organizations dealing with expansive data can rely on O.ai’s robustness for real-time analysis. Furthermore, its ability to integrate with popular big data platforms, such as Apache Spark, enhances its appeal. However, while O.ai shines in efficiency, users may find initial setup and configuration somewhat daunting without adequate technical knowledge.
Use Cases
Common use cases for O.ai range from credit scoring to fraud detection. Many financial institutions favor it due to its processing capabilities. For businesses focusing on predictive modeling, O.ai can provide substantial advantages. Yet, its effectiveness largely hinges on a team with solid statistical and machine learning foundations, which may be a limiting factor for less resource-abundant organizations.
Alteryx
Platform Overview
Alteryx presents itself as a data analytics platform that bridges the gap between data preparation and data analysis. It appeals broadly to business analysts and data scientists alike. Alteryx’s key characteristic is its drag-and-drop interface, simplifying complex data processes without deep data science knowledge. This can be particularly useful in a corporate environment where business users need access to data insights without heavy reliance on IT. However, such simplified usage comes at the cost of advanced analytic capabilities that some may find limiting.
Capabilities
Among its capabilities, Alteryx excels in data blending, helping users to combine data from various sources effortlessly. This translates into seamless workflows that keep data teams productive. The platform supports diverse data types, making it a versatile option across industries. The trade-off, however, is that its advanced analytics functionalities may not rival specialized tools designed strictly for machine learning.
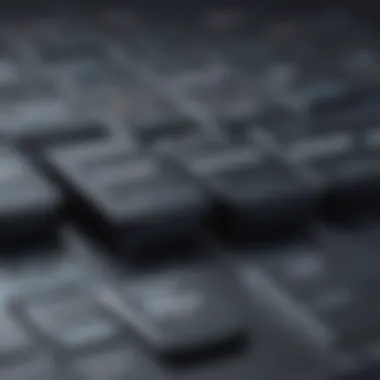
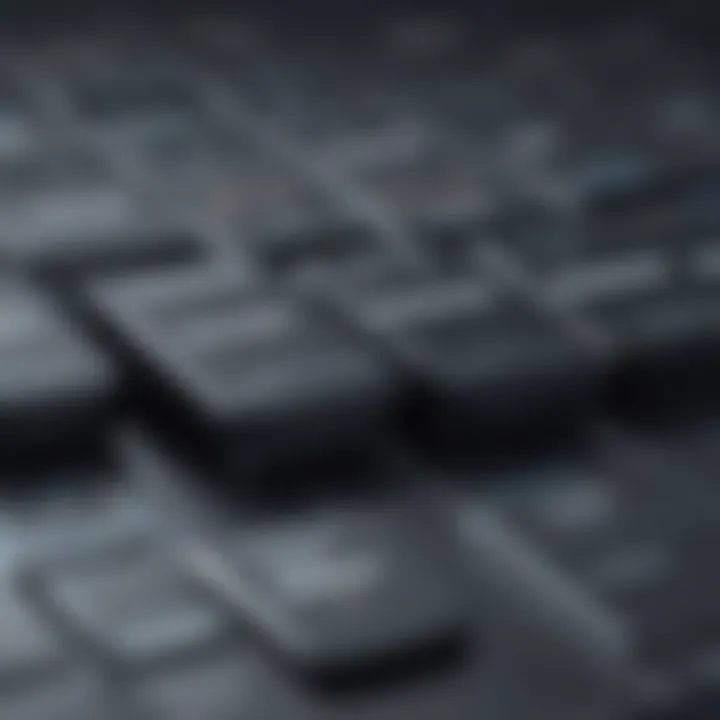
Market Positioning
Alteryx occupies a unique niche in the market — it empowers business users while still providing sufficient depth for seasoned data professionals. This positioning can be beneficial, as it allows companies to utilize existing team members for analytics tasks instead of sourcing specialized talent. Still, this compromise on depth might frustrate those looking for robust machine learning capabilities within the Alteryx framework.
RapidMiner
Preface to RapidMiner
RapidMiner stands out as a strong contender in the no-code data science space. The platform is designed to enable users to conduct advanced analytics without extensive programming experience. A defining characteristic of RapidMiner is its commitment to fostering a collaborative environment, allowing data scientists and domain experts to work together on projects seamlessly. While this collaboration can lead to insightful outcomes, it may necessitate some compromise in terms of speed, especially if teams aren't aligned.
Unique Selling Propositions
One of RapidMiner's unique selling points is its extensive library of algorithms and pre-built workflows. These pre-configured options save time and allow users to concentrate on refining their models rather than starting from scratch. Such features present rapid deployment capabilities which can be advantageous for businesses needing quick insights. However, reliance on pre-built models might not always cater to the specific nuances of unique datasets, limiting their applicability in specialized fields.
Target Audience
The target audience for RapidMiner widens to include both novice users and data enthusiasts seeking to understand machine learning better. The platform’s educational resources further attract learners looking to grow their data science skills. Nonetheless, while it caters to a broad audience, more advanced data scientists seeking cutting-edge capabilities might find RapidMiner somewhat lacking when compared to more sophisticated tools like O.ai.
Understanding these direct competitors provides valuable insights into the landscape. The efficiencies, user-friendliness, and capabilities of each platform contribute significantly to the decision-making processes of organizations looking for data science solutions.
Indirect Competitors and Alternatives
In the realm of data science platforms, understanding indirect competitors and alternatives to Domino Data Lab is akin to reading between the lines. These players may not directly challenge Domino’s offerings but still play a pivotal role in shaping the market landscape. This section sheds light on these alternatives, highlighting their importance and the unique benefits they bring to the table.
Indirect competitors often serve as valuable options for organizations seeking to optimize their data operations. They provide diverse functionalities and characteristics that cater to various business needs. Their presence amplifies the choices available to companies, especially small to medium-sized ones, that might be looking to harness data in innovative ways. Moreover, they can offer specific advantages, such as tailored pricing models or niche capabilities that direct competitors may overlook.
Microsoft Azure Machine Learning
Strengths and Weaknesses
One of the noteworthy aspects of Microsoft Azure Machine Learning is its robust integration with other Microsoft services. This feature provides a seamless experience for businesses already relying on Microsoft products. However, its complexity can be a double-edged sword. While the platform offers a broad array of tools for machine learning, it may overwhelm users who are new to data science.
This balance of strengths and weaknesses makes Azure a notable choice in this article. Its strong cloud architecture incorporates scalability and comprehensive analytics. Organizations value the ability to easily analyze vast datasets, but some find the learning curve steep, potentially leading to frustration.
Integrations
The integration capabilities of Azure are impressive. It can connect with numerous Microsoft tools and third-party applications, creating an ecosystem that simplifies workflows. For businesses entrenched in the Microsoft landscape, this is a crucial advantage. However, while this level of integration enhances collaboration, it can also create dependencies on Microsoft’s ecosystem, potentially locking companies out of exploring other viable options.
Google Cloud AI Platform
Key Features
Google Cloud AI Platform brings to the table powerful features rooted in Google's cutting-edge machine learning technology. Its autoML capabilities simplify the model training process, enabling even non-experts to design machine-learning models. This unique strength resonates well in a market that increasingly favors accessibility alongside capability. However, users may find that while the platform boasts impressive features, the cost can escalate quickly with extensive usage.
Cost Considerations
When it comes to cost, Google's platform is often seen as offering flexibility. The pay-as-you-go pricing model allows businesses to manage costs effectively but can also lead to unpredictability in expenses. Organizations need to carefully evaluate expected usage patterns against potential costs, making financial forecasting a bit of a balancing act.
IBM Watson
Product Overview
IBM Watson is recognized for its advanced capabilities in natural language processing and data analytics. Its ability to provide insights from unstructured data sets is particularly appealing for sectors like healthcare and finance. However, users might experience some limitations when it comes to user-friendliness; the learning process can be daunting. Despite these hurdles, Watson’s unique offerings make it a standout option within the data science tools realm.
Competitive Edge
What truly sets IBM Watson apart is its continuous investment in research and development. The platform stays relevant by regularly updating its capabilities, incorporating the latest advancements in AI and machine learning. This commitment to innovation is both a strength and a strategic advantage in a fiercely competitive market. However, some organizations may feel that the trade-off between performance and complexity can sometimes hinder its appeal.
"In the competitive landscape of data science, understanding the whole picture is essential for making informed software choices."
As businesses navigate through their various options, it becomes clear that indirect competitors and alternatives to Domino Data Lab have their own distinctive features and strategies. These insights guide organizations in making informed decisions that align with their specific needs.
Comparison Criteria for Data Science Platforms
When selecting a data science platform, there are a handful of key criteria that figure into the decision-making process. This article aims to draw attention to these factors as they significantly influence how an organization will interact with its chosen tools. Small to medium-sized businesses, entrepreneurs, and IT professionals are the main focus here because the right choice can make or break their projects.
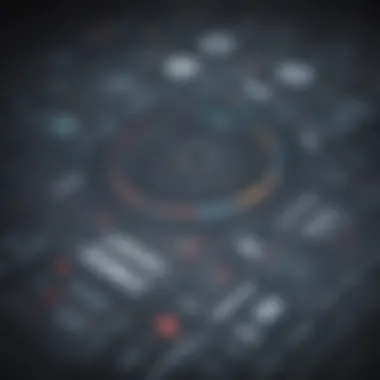
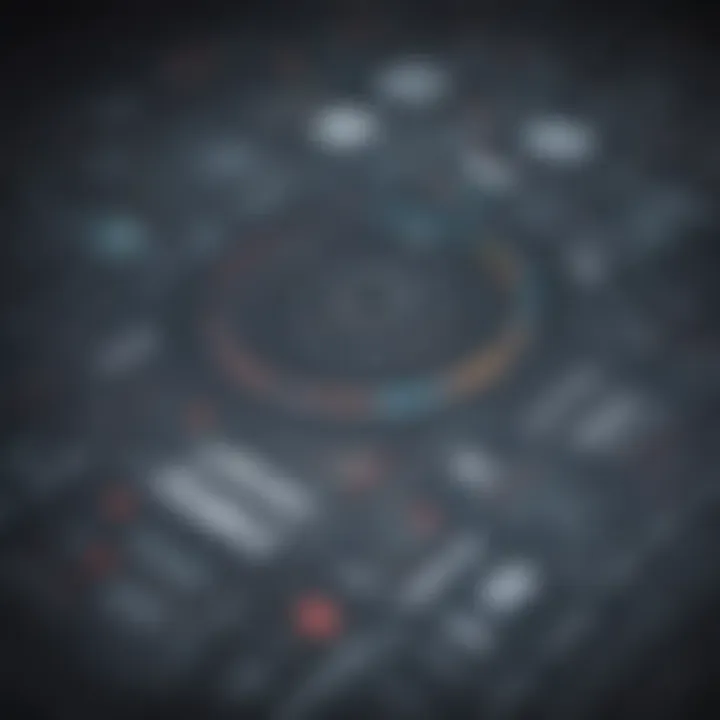
Understanding why these criteria matter helps in creating a clearer picture of what a platform can truly offer, going beyond just surface-level features. Because the landscape is constantly evolving, keeping these criteria at the forefront ensures organizations can navigate the options effectively while aligning with their unique needs.
Ease of Use
One of the most paramount criterion is ease of use. The interface should be intuitive enough that users don’t feel like they’re trying to decipher ancient hieroglyphics. After all, time is money, especially in the fast-paced world of data science. If a platform is clunky or requires excessive training, teams will likely hit a wall before they even get started.
The advantages of a user-friendly platform are manifold. A simplistic design grants users quick access to essential functions, ultimately speeding up the workflow and lending itself to more agile projects. Consideration should also be given to the level of technical knowledge required. For less tech-savvy staff, platforms that provide thorough tutorials or community forums can make the transition smoother. A platform with drag-and-drop functionalities often garners appreciation for its simplicity.
Scalability
Next in line is scalability. As businesses grow, their data science demands will undoubtedly evolve. A platform must be robust enough to accommodate increasing data loads without crumbling under pressure. Ideally, scalability ensures that the tools will support additional users and data types over time. This avoids the headache of migrating to a new platform when the existing one can no longer keep pace.
Moreover, a scalable platform should offer various tiers of services or modules—providing the flexibility to expand capabilities as needed. Cloud-based solutions often perform well in this regard, allowing businesses to easily upscale their operations without hefty overhead costs. Choosing a solution that can grow with the organization is a crucial consideration in today’s competitive environment.
Integration Capabilities
Now let’s dive into integration capabilities. Data science doesn't exist in a vacuum; rather, it interacts with numerous other systems. Seamless integration with existing databases, CRM systems, APIs, and BI tools is indispensable for effective data workflows. A platform that easily integrates with tools like Salesforce or Tableau will save considerable time and effort.
Moreover, the ability to work well with various programming languages—such as Python or R—also plays a crucial role in ensuring that teams can leverage the tools they are most familiar with. Proper integration guarantees the flow of information is both smooth and efficient, reducing the risk of data silos.
Support and Community
Last but definitely not least is support and community. Imagine trying to solve a significant issue only to find yourself in a sea of confusion, with no clear answers in sight. Good customer support can rescue teams from such predicaments. This means readily available help for troubleshooting, updates, and user inquiries.
Community forums can also add significant value. Users who experience common problems often band together to share solutions, tips, and best practices. A strong community spirit fosters innovation and can enhance overall user experience. Moreover, organizations should evaluate how frequently a platform releases updates and communicates with users about new features.
In summary, thorough consideration of ease of use, scalability, integration capabilities, and support can dramatically impact the effectiveness of data science platforms. Choosing wisely here creates a better environment for growth and innovation.
Future Outlook for Data Science Tools
As the landscape of data science continues to evolve, understanding the future outlook for data science tools is akin to having a crystal ball that reveals trends and capabilities yet to come. This section digs into why it's crucial for organizations, especially small and medium-sized businesses, to grasp these developments. Companies armed with insights about future tools and technologies can not only stay ahead of competitors but also make well-informed decisions that align perfectly with their business needs.
With constant advancements, the tools that companies use today might not suffice tomorrow. Businesses need to navigate a blend of predictive analytics, automation, and artificial intelligence, all of which are changing the fabric of data science. This evolving landscape impacts budget allocations, team capabilities, and even strategic initiatives.
Predicted Trends
The data science community is buzzing with predictions about which trends will take center stage. Here are some notable shifts that experts forecast:
- Increased Automation: Data science tools are expected to embrace automation, cutting down manual coding and allowing analysts to focus on interpretation rather than data cleaning.
- Ethical AI Development: As the public's awareness of ethical concerns regarding algorithms grows, the industry is moving towards implementing guidelines that ensure fairness in AI.
- Integration with Cloud Services: Tools will likely evolve to work seamlessly with cloud platforms, making it easier for businesses of all sizes to harness the power of big data without massive infrastructure investments.
- Continuous Learning: Models, instead of being static, will start to adapt on the fly as new data flows in, enabling businesses to gain real-time insights.
These trends not only indicate where we are headed but also highlight how data professionals must prepare. As one expert noted, "Navigating the future of data science tools requires an adaptable mindset and an eye on the horizon."
Technological Advancements
Technological advancements will play a pivotal role in shaping the data science toolbox of tomorrow. Here are a few key areas to watch:
- Machine Learning Enhancements: Algorithms will become increasingly sophisticated, leading to more accurate predictions and insights. Expect to see new methods that may outperform traditional models.
- Robust Data Processing: New techniques in processing will arise, enabling organizations to handle larger datasets more efficiently and swiftly. Apache Arrow and Dask are examples of platforms leading in this space.
- User-friendly Interfaces: Tools are making strides toward more intuitive user experiences. Aspects such as visual analytics will turn complex data manipulation tasks into drag-and-drop actions.
- Collaborative Features: Enhanced collaboration capabilities will emerge, breaking down silos within organizations. This will enable data scientists, engineers, and business stakeholders to work together fluidly on projects.
Making the Right Choice for Your Organization
Choosing the right data science platform can feel like navigating through a crowded marketplace with no signage. For organizations, particularly small to medium-sized businesses, this choice is not merely about selecting software; it's about aligning the technology with the actual needs of the business. The stakes are high, as the right decision can lead to improved operational efficiency, better data insights, and ultimately enhanced competitiveness. It's a significant investment, both in terms of money and time, so taking a thorough and thoughtful approach is crucial.
Assessing Business Needs
Understanding the unique requirements of your organization is the cornerstone of making an informed choice. It involves evaluating what specifically your business aims to achieve with data science tools. For example, are you mainly focused on predictive analytics, or is exploratory data analysis more in line with your goals? Different platforms excel in different areas, and aligning these strengths with your business goals can mean the difference between success and a missed opportunity.
Key considerations include:
- Current Data Infrastructure: Assess what existing systems and software are in place. Are they scalable? Can new tools integrate seamlessly with them?
- Use Cases: Identify the primary scenarios you want to address. Whether it’s customer segmentation, sales forecasting, or operational optimizations, understanding these use cases will direct your search.
- User Skill Level: Evaluate the skills present in your team. Bringing in a sophisticated tool without the necessary expertise can inhibit success.
- Budget Constraints: Be real about what you can spend while considering both direct and indirect costs associated with various platforms.
Once these factors are clear, you can begin to narrow down your options, making the choice less overwhelming.
Cost-Benefit Analysis
Once you’ve done the groundwork of assessing your needs, it’s time for a deep-dive into the financial implications of your choices. A thorough cost-benefit analysis allows decision-makers to weigh up not just the initial investment but also ongoing costs and potential return on investment.
Performing this analysis involves looking at:
- Software Licensing Fees: Often the most straightforward cost, this includes upfront fees and any monthly or annual subscriptions that may apply.
- Integration Costs: If the chosen platform doesn’t play nicely with other software, you might need to invest in additional tools or custom development.
- Training and Support: Budget for training your team and any ongoing support you may need to address issues as they arise.
- Opportunity Costs: Consider what your organization might miss out on if the new platform doesn’t deliver as expected or consumes more resources than anticipated.
A well-rounded cost-benefit analysis helps paint a clear picture of economic implications and can make it easier to justify the decision to stakeholders or financial backers.
"The right tools act as force multipliers in data science, amplifying the impact of your team’s efforts while helping to keep costs in check."