Top Google BigQuery Alternatives for Data Solutions
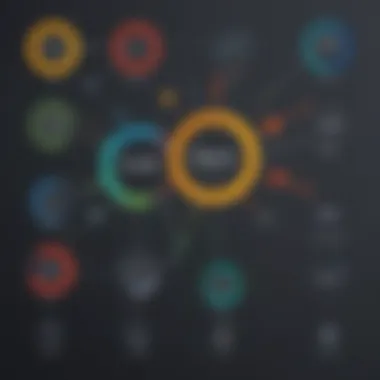
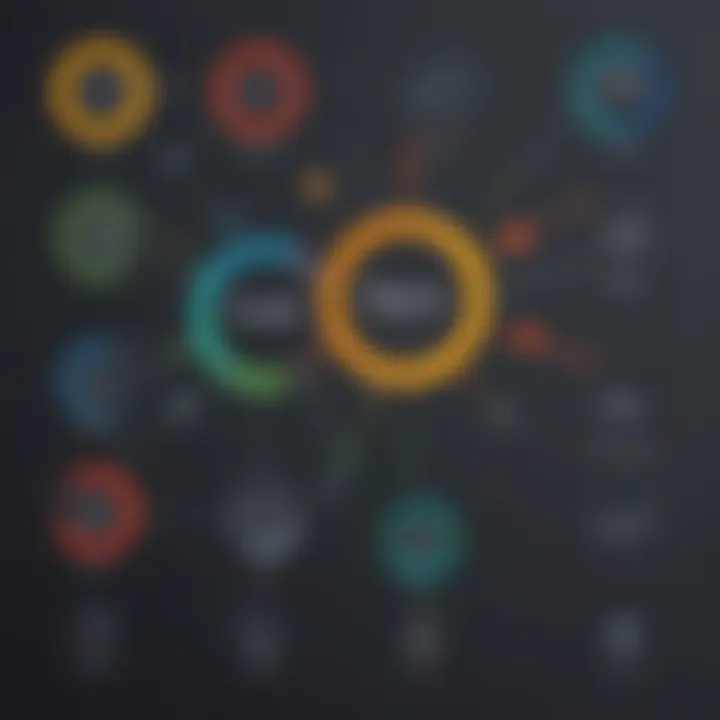
Intro
In today’s rapidly changing data ecosystem, organizations are increasingly recognizing the importance of efficient data processing and management solutions. Google BigQuery has positioned itself as a leader in this arena, offering powerful capabilities for analytics at scale. However, as the landscape evolves, various alternatives emerge that present themselves as viable options for those seeking flexibility, cost-effectiveness, or specific features suited to particular business needs.
This article will examine several alternatives to Google BigQuery, providing insights into their core functionalities, costs, and ideal use cases. Whether you are a small business owner looking to maximize a tight budget, an IT professional tasked with finding the right tools, or an entrepreneur eager to explore how different solutions stack up, this analysis aims to serve as a stepping stone to informed decision-making.
By closely analyzing these options, we delve into both the advantages and limitations each solution presents. Whether it's agility, scalability, or storage costs, understanding the nuances of each choice plays a crucial role in tailoring the right strategy for your data management needs.
Key Features of the Software
Harnessing the strengths and unique functionalities of different tools can lead to more tailored data solutions. We will explore the alternatives available and what they bring to the table.
Overview of Core Functionalities
- Amazon Redshift: This is a popular data warehouse service that excels in large-scale data analysis. It offers features such as consistent performance, high query speeds, and seamless scaling options.
- Snowflake: This platform prides itself on its cloud-native architecture, separating storage and compute to give users flexibility. Notably, Snowflake supports diverse data types, making it suitable for various analytical needs.
- Microsoft Azure Synapse Analytics: Formerly known as Azure SQL Data Warehouse, this service combines data integration, big data analytics, and enterprise data warehousing. Its real-time analytics capabilities are worth noting for businesses looking for immediacy in insights.
Unique Selling Points
- User Experience: Some solutions prioritize straightforward interfaces that allow teams to work without extensive training. This can be crucial for smaller businesses with limited resources.
- Integration Capabilities: The ability to integrate seamlessly with existing systems or workflows is a significant advantage for organizations looking to streamline operations.
- Cost Structure: Different approaches to pricing can align better with business models. For instance, Snowflake offers a consumption-based pricing model that can significantly lower costs for businesses with fluctuating workloads.
In-Depth Software Analysis
Understanding the advantages and drawbacks of each option can empower decision-makers to choose wisely.
Pros and Cons
Amazon Redshift
Pros:
- High performance for complex queries
- Scalable to petabytes of data
Cons:
- Requires more upfront management
- Costs can escalate with a high volume of queries.
Snowflake
Pros:
- Offers pay for use model, making it cost-efficient
- Easy to set up and manage
Cons:
- The unique architecture may not suit every organization.
Microsoft Azure Synapse Analytics
Pros:
- Strong integration with other Microsoft products
- Real-time analytics for timely insights
Cons:
- May be overwhelming for users unfamiliar with Azure ecosystem.
Performance Metrics
Performance can be subjective based on specific needs, but certain metrics can guide expectations:
- Query Speed: How long it takes for requests to return results.
- Scalability: The ability to handle increasing amounts of data without service interruption.
- Cost-efficiency: Evaluating the total cost of ownership based on usage patterns.
Exploring these performance metrics not only ensures the right fit but can lead to significant operational efficiencies, helping businesses leverage their data for strategic advantage.
Prologue
In the rapidly evolving landscape of data management and analysis, small to medium-sized businesses, entrepreneurs, and IT professionals find themselves at a critical juncture. The efficiency and effectiveness of their data processing systems can make or break their strategic initiatives. Google BigQuery has made a significant mark in this arena, offering powerful capabilities for handling vast amounts of data. However, as much as it shines, it's not a one-size-fits-all solution.
Exploring alternatives to Google BigQuery isn't just an academic exercise; it's a necessity in the current age of information overload. The right choice can lead to better performance, cost savings, and ultimately superior business insights. Each available option brings its own set of features and limitations that must be examined closely.
Understanding deviations from BigQuery’s model can illuminate paths that are more suitable for the unique challenges faced by different businesses. For instance, a company’s data needs may shift based on seasonal demand or evolving project requirements. Users may seek increased customization, scalability, or improved integration capabilities, which calls for a comprehensive analysis of the alternatives.
Key Considerations
The decision to explore alternatives hinges on various factors:
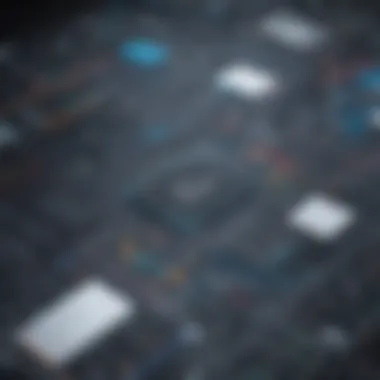
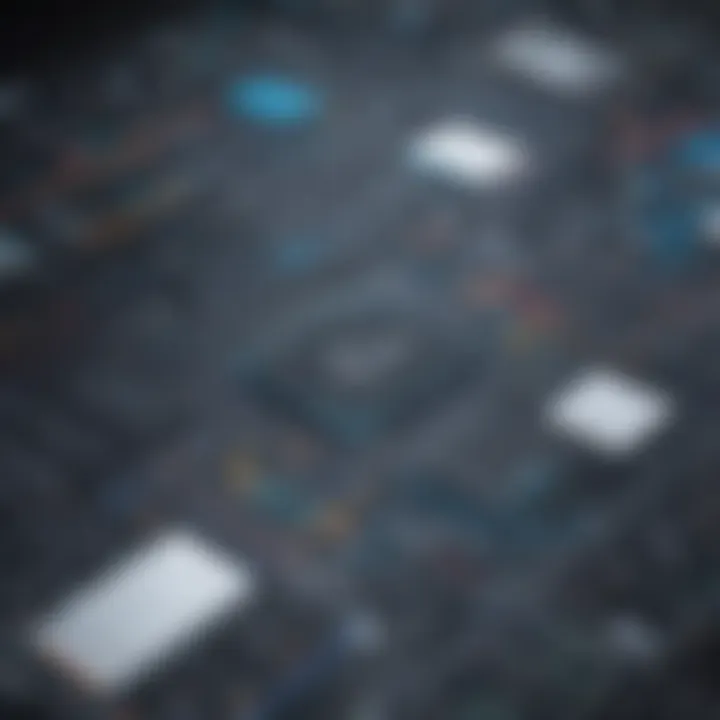
- Customization Needs: Different businesses have unique demands. A solution that offers flexibility can adapt to various use cases and workflows.
- Cost Considerations: In today's economy, every penny counts. Understanding the pricing structures of alternatives can mean the difference between a sustainable long-term solution versus a costly blunder.
- Performance Metrics: Speed and reliability in data processing can vary significantly across platforms. Knowing which option stands up to scrutiny is paramount.
"Choosing the right data processing solution is like finding the perfect partner; you must consider compatibility, costs, and overall fit with your goals."
In summary, embarking on this examination of Google BigQuery alternatives is not simply about finding products. It's about understanding what fits best for specific operational needs in a competitive landscape. Engaging with this topic prepares businesses to make informed decisions that weigh their particular requirements against the offerings available in the market.
Overview of Google BigQuery
In today’s fast-paced data-driven environment, understanding the utility of platforms like Google BigQuery is paramount for businesses keen on making informed decisions with their data. BigQuery is Google’s fully-managed, serverless data warehouse, designed to enable the quick and efficient analysis of massive datasets. Its prowess lies in handling large volumes of data with seamless integration into other Google Cloud services.
This section covers core features, common use cases, and the limitations of BigQuery. Knowing these elements is crucial for any business or IT manager considering their data processing solutions, as they reflect not just the capabilities of BigQuery, but frame the context from which alternatives are assessed.
Core Features
Scalability and Speed: BigQuery stands out mainly due to its ability to handle massive datasets, scaling effortlessly. It can query petabytes of data in seconds, which is a serious advantage in the realm of big data analytics.
Serverless Architecture: The serverless nature eliminates the need for complex infrastructure management, thus allowing organizations to focus purely on analysis rather than setup and maintenance.
SQL Compatibility: Most data analysts and scientists are familiar with SQL, making BigQuery’s SQL-like interface highly user-friendly. This aspect assists teams in transitioning smoothly into utilizing the platform without extensive retraining.
Common Use Cases
Business Intelligence: Many organizations leverage Google BigQuery for reporting and data visualization. Tools like Tableau and Looker easily integrate with it, allowing stakeholders to derive actionable insights effortlessly.
Machine Learning Models: BigQuery Machine Learning (BQML) allows users to create and execute machine learning models directly in SQL. This provides a way for analysts to harness predictive analytics without needing deep knowledge of coding languages, actually bringing the power of ML to the data analyst's fingertips.
Log Analysis: Companies often use BigQuery for analyzing logs from various applications and services. Its ability to perform queries over large log data quickly means businesses can troubleshoot issues and monitor operations effectively.
Limitations
Despite its advantages, there are significant limitations to Google BigQuery that organizations must weigh against its benefits:
Cost Considerations: While the pay-as-you-go model can be beneficial at first glance, query costs can spiral quickly for large datasets, particularly if not managed properly. Companies may find themselves facing unexpected charges.
Data Transfer Issues: For businesses with data not already in the Google ecosystem, transferring substantial datasets can be cumbersome and time-consuming. This can cause delays in the implementation of BigQuery as a solution.
Lack of Advanced Features: Compared to some competitors, BigQuery may lack certain advanced data manipulation and analytical features, limiting the scope for those with specific needs in complex data scenarios.
"Evaluating Google BigQuery is critical to understanding its place among other data solutions, particularly for businesses navigating their unique data landscapes."
This overview of Google BigQuery lays the groundwork for understanding why businesses might seek alternatives to this powerful but sometimes limiting data analysis tool. By delving deeper into the alternatives, such as Amazon Redshift or Snowflake, we can appreciate how each platform addresses specific organizational needs.
Importance of Exploring Alternatives
When it comes to data analytics, relying solely on a popular platform like Google BigQuery might not be the best fit for every organization. Often, the need for exploring alternatives stems from specific operational requirements and financial constraints. BigQuery undoubtedly offers a slew of powerful tools; however, diving deeper into alternatives can reveal solutions that better align with unique business needs.
Customization Needs
Every business is a different fish in the sea. What suits one outfit may not suit another. Customization has become a cornerstone of modern software solutions. While Google BigQuery provides a robust environment for analytics, some organizations may find its features somewhat rigid for unique data workflows. For instance, a startup focused on niche market analysis might need finer control over queries and data models than BigQuery allows. On the flip side, alternatives like Snowflake offer data sharing capabilities and are designed for more tailored data handling, making it a more fitting choice for businesses with specific needs.
Customization isn’t just about features; it’s also about adjusting the user experience to harmonize with existing processes. It’s like trying on shoes; the perfect fit varies from person to person. Whether you require an integrated toolset, user-defined workflows, or specialized data pipelines, alternatives can provide solutions that resonate with your operational style.
Cost Considerations
In the realm of data analytics, what you see isn’t always what you get—especially when it comes to costs. For small to medium-sized businesses, understanding the pricing structures of data solutions is a pivotal factor in decision-making. Google BigQuery operates on a pay-as-you-go model, which can be an attractive option at first glance but can potentially escalate quickly based on usage patterns.
Alternatives like Amazon Redshift or Microsoft Azure Synapse offer varied pricing structures that might suit different budgets better. It’s crucial to analyze these costs from every angle. Consider not just the direct expenses but also the long-term implications on subscription fees, storage needs, and processing costs. In the end, choosing a platform that maintains financial sustainability while delivering efficacy is paramount.
Performance Metrics
Performance is like the beating heart of data analytics operations. A platform’s ability to handle queries, process data, and deliver insights promptly can significantly influence business agility. Google BigQuery enjoys a reputation for speedy processing times, yet that doesn’t mean other solutions aren’t running a close race.
For instance, Apache Spark shines in its data processing speed, particularly for big data applications. However, it comes with its own set of complexities, particularly when it comes to setup and maintenance. Therefore, assessing performance metrics is not just about raw speed; it’s also about understanding how well a platform integrates with your existing architecture, as well as its ability to scale as needs grow.
"Performance is not a feature; it’s a necessity."
Each alternative brings unique strengths and weaknesses to the table. Carefully aligning these with business objectives can offer profound insights into which solution might serve your team best.
Exploring alternatives to Google BigQuery is not merely an exercise in variety; it’s a strategic move that can unlock potential benefits in customization, cost, and performance. Each organization stands to gain significantly from reviewing their options and finding the right tool for their unique data landscape.
Alternative Solutions to Google BigQuery
In the fast-paced world of big data analytics, reliance on a single platform can be like putting all your eggs in one basket. As companies seek to harness their data for actionable insights, the importance of exploring alternatives to Google BigQuery cannot be overstated. There are numerous players in the market, each offering distinct features, benefits, and tailored pricing structures that can align better with specific business needs. By examining these alternatives, organizations can optimize their data strategies to suit their growth trajectories.
Amazon Redshift
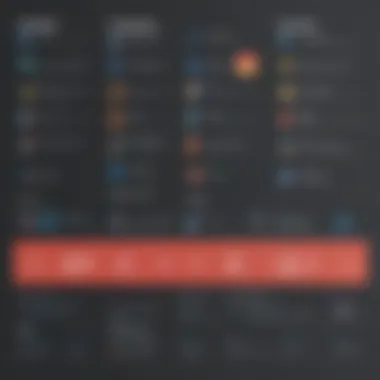
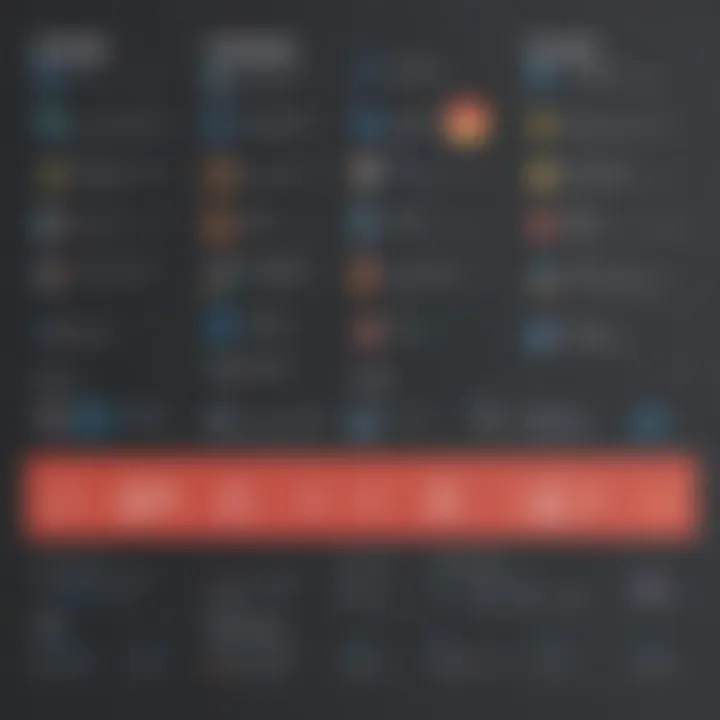
Features Overview
Amazon Redshift is likened to a robust vault for data. Its notable characteristic lies in its architecture optimized for complex queries and high-performance analytics. The use of a columnar storage model allows it to compress data efficiently, making it a beloved choice for users aiming to reduce query costs while enhancing performance. One unique feature of Amazon Redshift is its ability to scale up and down effortlessly based on workload demands, a crucial element for businesses that experience fluctuating data usage.
Pricing Structure
When delving into the pricing structure of Amazon Redshift, it’s essential to highlight the pay-as-you-go model. Users only pay for what they consume, which allows companies to manage budgets more effectively. Additionally, based on its tiered offerings, users can choose different instance types that suit their performance needs without breaking the bank. However, some may find the costs can mount up, especially with high storage needs, so close monitoring is adviced.
Performance Insights
Performance insights speak volumes in data analytics. A standout characteristic of Amazon Redshift is its ability to handle petabytes of data while still providing sub-second response times for queries. The result? Businesses can scale their data operations without sacrificing speed or efficiency. Unique to Amazon Redshift is the mix of machine learning capabilities built into query optimization, which provides users with an intelligent analytics solution that continuously improves performance. Nonetheless, as with most tools, underestimating the need for proper maintenance and optimization could lead to slowdowns.
Snowflake
Data Sharing Capabilities
One of the key selling points of Snowflake is its powerful data sharing capabilities. Users can easily share data across departments and with external partners without duplicating datasets. This feature promotes real-time collaboration, a critical aspect for data-driven decision-making. The unique architecture of Snowflake, designed for concurrent usage with no data contention, solidifies its reputation as a go-to tool for businesses focused on agility.
Scalability Options
In terms of scalability, Snowflake takes the cake. Its auto-scaling functionality means that resources can be allocated seamlessly without any downtime, ensuring that analytics processes are never slowed down by resource limits. This elasticity allows companies to adjust based on their needs, whether during peak periods or low activity times. However, the necessity for a clear understanding of how scaling impacts costs can be a hurdle for some users seeking best value.
Competitive Analysis
Analyzing the landscape, Snowflake’s position is more pronounced than ever. Its architecture is built on cloud-native technology, which differentiates it from older models. Furthermore, the competitive analysis shows Snowflake consistently edges out on user satisfaction, especially among those needing real-time insights. Nevertheless, navigating its somewhat convoluted pricing can be a barrier for smaller enterprises looking to adopt it.
Azure Synapse Analytics
Integration with Azure Services
The importance of integration with Azure services cannot be understated for businesses already embedded in the Microsoft ecosystem. Azure Synapse Analytics offers seamless connections to various Azure products, enhancing workflows and improving data handling efficiencies. This characteristic enables users to leverage existing investments while expanding their analytic capabilities. As a unique feature, users can integrate data pipelines effortlessly, strengthening the overall architecture required for robust data systems.
Collaboration Tools
Collaboration tools within Azure Synapse are designed to facilitate teamwork. With integrated features allowing multiple users to work on projects simultaneously, it stands out as a beneficial choice for teams needing real-time collaboration. A unique aspect is the ability to share insights directly through Azure dashboards, helping teams stay aligned on critical business metrics. The downside? Teams must be comfortable navigating Azure's sometimes complex interface, especially for new users.
Cost vs. Value
On the topic of cost vs. value, Azure Synapse shines for companies already familiar with Microsoft's pricing structure. Its flexibility provides a significant value proposition, offering various tiers depending on usage needs. However, potential clients should weigh whether the investment aligns with their specific analytic needs, as misalignment can lead to unnecessary expenditure.
Apache Spark
Open-Source Benefits
Apache Spark stands as a pinnacle of open-source solutions in data processing. One critical aspect is the fact that it provides broad versatility and flexibility, enabling businesses to tailor their environments according to specific needs. A key characteristic of Spark is its ability to process large volumes of data swiftly and efficiently. This adaptability may, however, require companies to invest in technical training, which can be a disadvantage for businesses lacking data engineering resources.
Data Processing Speed
When it comes to data processing speed, Apache Spark often outperforms many competitors. Its in-memory computation provides substantial speed advantages, allowing it to handle real-time data streams effectively. This capacity can open up a whole world of possibilities for businesses seeking to harness big data quickly. However, scalability concerns may arise as processing loads increase, demanding careful resource management.
Community Support
Community support is another strong aspect of Apache Spark. Being open-source means that users have access to a community of contributors which can sometimes lead to innovative solutions not officially supported. However, the reliance on community-driven updates can feel inconsistent for organizations looking for formally guaranteed support. Still, the shared wealth of knowledge can be invaluable for troubleshooting and optimization.
IBM Db2 Warehouse
Hybrid Deployment Options
IBM Db2 Warehouse offers a remarkable hybrid deployment option, allowing businesses to run their databases both on-premises and in the cloud. This flexibility is essential for enterprises managing sensitive data that cannot consistently reside in the cloud. A key characteristic of this system is its semi-automated processes that ease deployment headaches. However, such hybrid solutions can lead to increased complexity if not managed properly.
AI and Machine Learning Features
Incorporating AI and machine learning capabilities into its environment, IBM Db2 Warehouse gives users the ability to streamline analytics with predictive tools. This is increasingly becoming a necessities in today's data-centric world. Organizations gain unique advantages by being able to derive complex insights without requiring extensive coding skills. The flip side? Companies must ensure they have a nuanced understanding of AI applications to avoid misinterpretations.
User Feedback
The user feedback surrounding IBM Db2 Warehouse often highlights performance stability and reliability. Businesses see value in its consistent outcomes, though this tool has also been critiqued for having a steeper learning curve compared to its counterparts. Thus, organizations must commit time for proper training before fully leveraging its capabilities.
ClickHouse
Real-Time Analytics Capabilities
For businesses focusing on real-time analytics, ClickHouse presents an attractive option. Its inherent design radically optimizes for speed and allows users to ingest data in real-time without delays. This characteristic caters to rapidly changing business environments where timely data utilization is vital. However, dealing with such speed may require additional resource allocation, as systems must be reinforced for continual upkeep.
Integration Possibilities
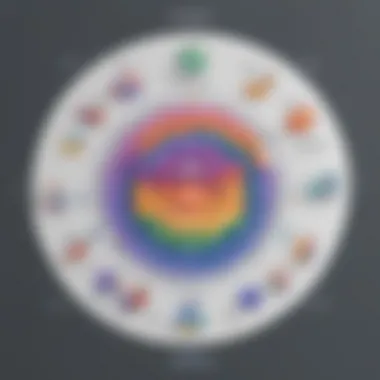
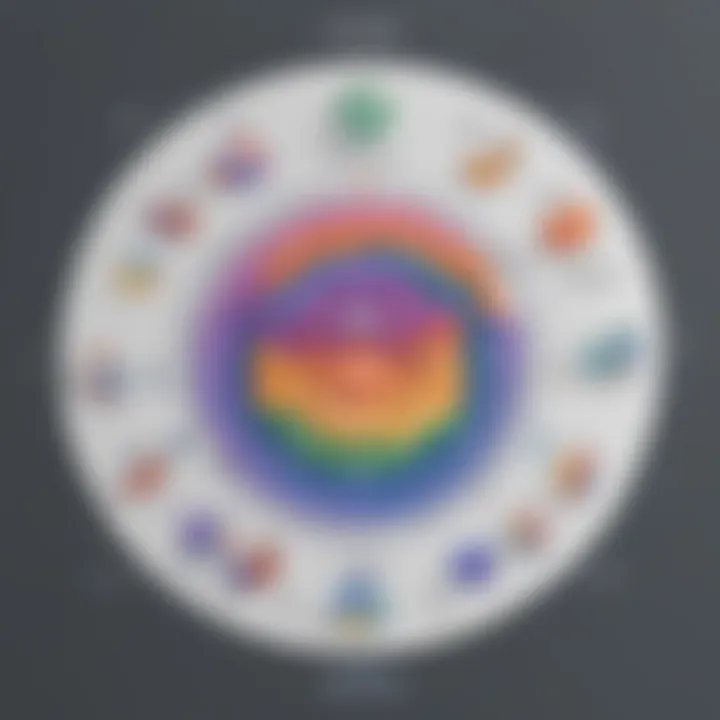
The integration possibilities with ClickHouse are broad—allowing it to connect seamlessly with popular querying languages and frameworks. This characteristic of fluid compatibility makes it a suitable choice for varying use cases across industries. Nonetheless, the potential learning curve associated with its specific querying syntax might deter some users initially.
Use Case Scenarios
ClickHouse thrives in various use case scenarios, especially those needing immediate insights, such as eCommerce and online marketing analytics. Its ability to swiftly process complex queries places it ahead in situations demanding agility and precision. Just remember, while it rationalizes immediate needs, overdependence without solid support structures may lead to challenges in the longer run.
Oracle Autonomous Data Warehouse
Automation and Self-Tuning Features
The hallmark of Oracle Autonomous Data Warehouse is its smart automation. Users appreciate the self-tuning features that continuously optimize operations without manual intervention. This capability streamlines routine tasks, allowing data teams to focus on larger strategic initiatives rather than day-to-day maintenance. Yet, organizations should weigh this efficiency against the complexity of implementing such sophisticated systems, which can be a barrier for smaller firms.
Security Measures
Security is a top priority, especially in today's data-driven age. Oracle takes this seriously by embedding robust security measures into its Autonomous Data Warehouse. Strong encryption and user access controls are vital highlights. However, the challenge lies in ensuring that users understand how to configure these security features properly, to avoid vulnerabilities.
Market Positioning
In the competitive landscape, Oracle’s Autonomous Data Warehouse positions itself strongly among enterprise solutions. Its focus on both user experience and powerful analytics capabilities makes it appealing for larger organizations. Despite this, smaller businesses may find it overwhelming, and thus need to assess whether the system matches their needs.
Criteria for Selecting an Alternative
When considering alternatives to Google BigQuery, it's crucial to establish criteria for selection. This process ensures that businesses find a data solution that aligns perfectly with their unique needs. Evaluating alternatives involves examining various factors that can impact an organization's efficiency, effectiveness, and ability to leverage data insights. The criteria for selecting an alternative are not just technical; they encompass operational, financial, and strategic considerations.
Data Volume Handling
One of the foremost considerations is how well an alternative can manage data volume. Different businesses experience different data loads, and the solution must align with these volumes. Just imagine a retail chain with a vast influx of transactions during the festive season versus a small startup with modest, steady growth.
A viable alternative should effectively scale for large datasets, permitting seamless querying and analysis without sacrificing performance. The capacity for handling massive datasets is paramount, particularly for businesses in sectors like finance or healthcare, where compliance and accuracy are integral. It’s advisable to look into:
- Scalability: How does the platform adapt as the data grows?
- Load Time: What is the average time required to ingest large batches of data?
- Storage Options: Is there flexibility in storage for structured and unstructured data?
Query Performance
Query performance plays a critical role in determining user satisfaction and overall productivity. In the world of data analytics, a few seconds saved in querying can lead to substantial time savings across the board. Businesses can’t afford to miss insights simply because a query takes too long to process.
The alternative solution should offer:
- Speed of Query Execution: Evaluate benchmarks or case studies that exhibit its performance.
- Optimized Queries: Does the platform provide optimization tools to improve run times?
- Concurrent Query Processing: Can multiple users execute queries at once without slowdowns?
A platform’s query performance will directly influence how insights are derived, making this a vital area of consideration.
User Accessibility
User accessibility transcends just enabling users to log in; it's about ensuring every level of user—from technical teams to business stakeholders—can utilize the platform effectively. A robust alternative should feature intuitive interfaces that reduce the learning curve, especially for those less technically inclined.
Consider the following:
- User Interface Design: Is it user-friendly and easy to navigate?
- Training Resources: Are there adequate educational materials provided, like tutorials or documentation?
- Role-Based Access: Can permissions be tailored to suit different roles within a business?
When users feel empowered rather than frustrated, the whole organization benefits from better decision-making through data.
Integration Compatibility
Integration capabilities cannot be overstated. In today's ecosystem, businesses typically rely on multiple tools to manage data. An alternative must effortlessly integrate with existing software to maintain workflow efficiency and maximize data value.
Key points to investigate include:
- API Availability: Does the alternative provide APIs for a smooth connection to other systems?
- Third-Party Tools: How well does it integrate with analytics tools, CRM systems, or cloud platforms?
- Data Migration Support: What support is available for migrating data from existing systems?
Choosing a platform that fits seamlessly into the current tech landscape can lead to substantial time and cost savings.
"Each business’s data needs are unique—selecting the right analytic tool can determine how effectively it competes in the marketplace."
Ending
The conclusion serves as a crucial touchpoint in encapsulating the insights gathered throughout this article. In a landscape dominated by vast data needs, understanding Google BigQuery alternatives is not merely an academic exercise; it's a business imperative. The need to explore these alternatives arises from multiple factors that can significantly shift a company's capabilities in handling data analytics.
Summarizing Key Insights
It is essential to reiterate some key takeaways. First, the diversity in available alternatives—ranging from Amazon Redshift to ClickHouse—offers organizations tailored features suited for various types of data tasks. Each platform has its own strengths:
- Amazon Redshift shines through with scalability, fulfilling the needs of businesses experiencing rapid data growth.
- Snowflake provides exceptional data sharing capabilities, making it a favorite among teams needing to collaborate effectively without duplicating data.
- Azure Synapse Analytics integrates seamlessly with Azure services, which could be a game changer for users already invested in that ecosystem.
- Apache Spark's open-source advantage allows businesses to harness community-driven innovation for enhanced data processing speeds.
Understanding these insights empowers decision-makers to tailor their data strategies effectively. It's about matching the right tool to the right job, ensuring optimal performance at a cost that justifies the investment.
Future Outlook for Data Analytics Solutions
Looking ahead, the field of data analytics is evolving, with newer technologies sprouting up regularly. As organizations pursue insights that drive meaningful actions, they will increasingly recognize the value of alternatives offering specific features geared toward their unique requirements.
The current trend suggests a more integrated approach to data, combining capabilities from multiple platforms to build hybrid solutions. Expect a future where:
- Interoperability becomes the norm, allowing systems to communicate smoothly.
- AI integration will further enhance analytics, enabling predictive modeling and real-time insights.
- Cost efficiencies will arise as competition among data platforms intensifies.