Understanding Azure Databricks Pricing for Businesses
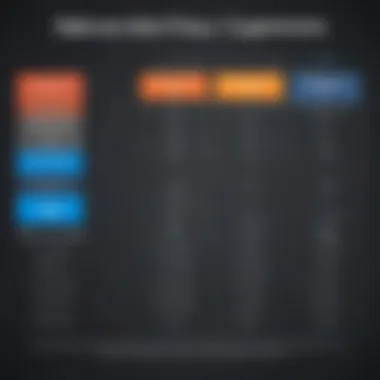
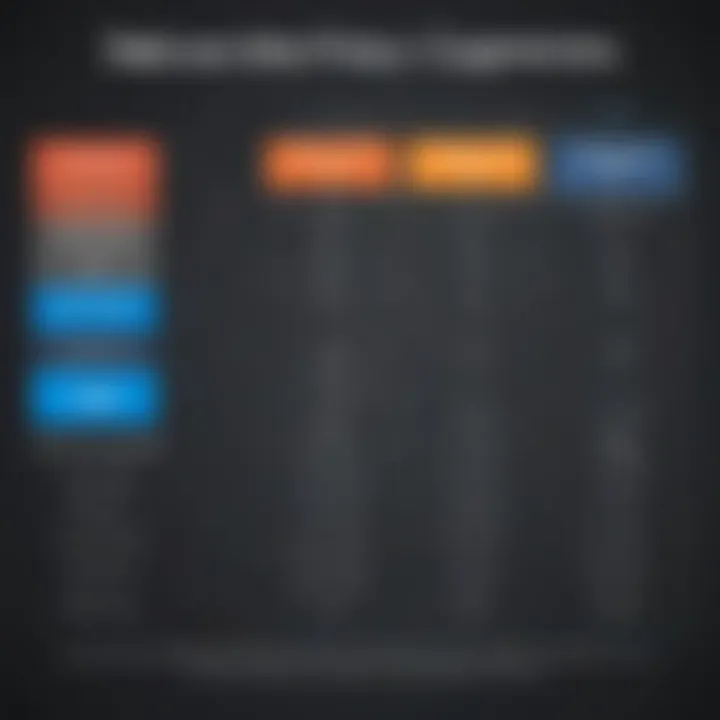
Intro
Azure Databricks offers a powerful platform tailored for big data and data science. Understanding the pricing model is crucial for businesses seeking to maximize their investments in cloud analytics. This article will unfold various aspects of Azure Databricks pricing, including its core functionalities, common pricing models, and comparison with other solutions. It provides insights not only into cost factors but also into performance metrics, thus allowing enterprises to make informed decisions regarding their cloud analytics expenditures.
Key Features of the Software
Azure Databricks has several key features designed to support data processing and analytics. These features include:
- Unified Analytics: Combines data engineering and data science workflows. This integrative approach promotes collaboration among different teams.
- Collaborative Notebooks: Allows data scientists and engineers to share insights seamlessly using notebooks that support real-time collaboration.
- Optimized Apache Spark Runtime: Azure Databricks provides an optimized runtime for Apache Spark. This will enhance the performance of big data processing tasks.
- Built-In Machine Learning Support: The platform offers features to streamline machine learning workflows, making it an attractive option for organizations looking to implement advanced analytics.
Overview of Core Functionalities
The functionalities central to Azure Databricks include data preparation, model training, and deployment. This allows organizations to easily transition from raw data ingestion to actionable insights. Furthermore, integration with various data storage services adds to its flexibility, accommodating diverse enterprise architectures.
Unique Selling Points
One of the unique selling points of Azure Databricks lies in its scalability. As business needs grow, the platform can adjust resources accordingly. Additionally, the integration with Microsoft Azure ensures compatibility with a suite of services, enabling companies to leverage existing investments in Azure technologies.
In-Depth Software Analysis
Delving deeper into Azure Databricks reveals both its strengths and weaknesses.
Pros and Cons
Pros:
- Scalable Architecture: Businesses can allocate resources as needed, avoiding unnecessary costs.
- High Performance: The platform's optimization leads to faster processing times. This can lead to improved productivity.
- User-Friendly Interface: Its interface simplifies complex tasks, making it accessible for professionals across skill levels.
Cons:
- Complex Pricing Structure: Understanding the pricing can be challenging, especially for new users who may struggle with various cost components.
- Dependency on Azure: Organizations heavily reliant on non-Azure ecosystems may find integration more cumbersome.
Performance Metrics
Performance is key when considering any analytics platform. Azure Databricks shows impressive metrics, particularly in speed and processing capacity. Its Spark-based architecture enhances throughput for large datasets. Moreover, real-time data processing capabilities support businesses that require immediate insights.
Azure Databricks' performance excels in speed and scalability, vital for enterprises dealing with large data workloads.
Through this comprehensive guide, readers will better understand the multifaceted pricing model and the precise elements influencing costs on Azure Databricks.
Overview of Azure Databricks
Understanding Azure Databricks is crucial for businesses seeking to harness the power of big data analytics. This platform integrates seamlessly with Azure services, enabling users to perform large-scale data processing while leveraging the advantages of Apache Spark. With the increasing demand for analytics in business operations, companies aim to extract deeper insights from their data. Thus, a clear grasp of how Azure Databricks operates can lead to more informed decisions regarding its implementation and pricing.
Importance of Azure Databricks
The significance of Azure Databricks lies in its ability to simplify data workflows. Organizations can efficiently analyze structured and unstructured data using diverse tools without needing complex configurations. As a collaborative environment, it allows data engineers, data scientists, and business analysts to work together in real-time.
Benefits to Organizations
Several benefits emerge when organizations utilize Azure Databricks:
- Scalability: Databricks offers users the capacity to scale resources according to demand. Businesses only pay for what they use, which lessens the risk of overspending.
- Integration: It connects effortlessly with various Azure services, such as Azure Data Lake Storage and Azure Machine Learning. This integration fosters a robust ecosystem for analytics.
- Speed: Built on Apache Spark, Azure Databricks enables faster data processing tasks compared to conventional methods. This efficiency can lead to quicker insights and decision-making.
Considering these factors, understanding the intricacies of Azure Databricks becomes paramount. Organizations that prioritize comprehensive insight into pricing structures, features, and potential applications will navigate their cloud analytics journey more effectively.

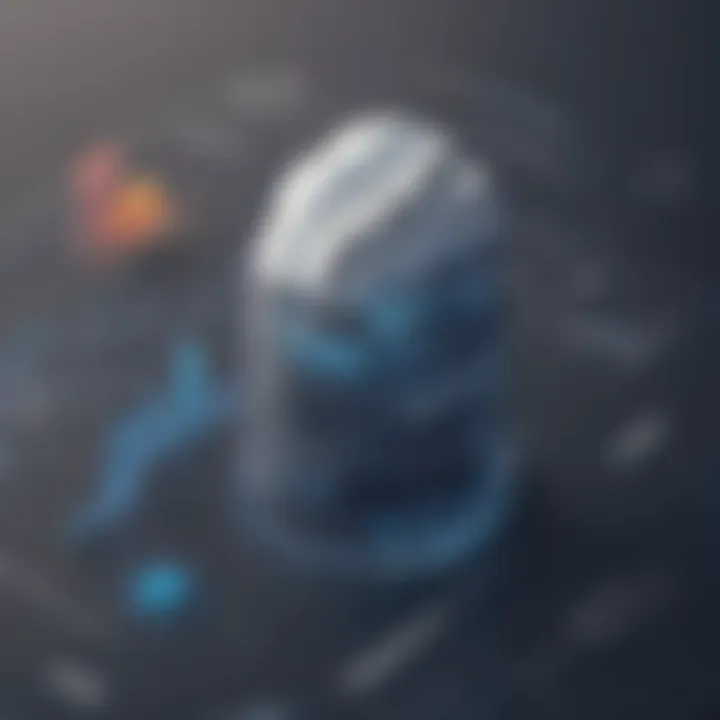
Azure Databricks Pricing Structure
Understanding the pricing structure of Azure Databricks is crucial for businesses considering its adoption. A clear grasp of costs can lead to better budget planning and optimized resource allocation. Without understanding, companies may face unexpected financial burdens, which can complicate the deployment of this sophisticated analytics platform.
The Azure Databricks pricing structure consists of multiple components that can vary significantly based on usage patterns and resource types. Each component plays a role in determining what the final billing will look like. Businesses need to evaluate their specific needs against these components to forecast expenses accurately.
Components of Pricing
Azure Databricks pricing comprises several underlying components. These components include:
- Compute Costs: This is most significant in terms of pricing. Databricks uses clusters of virtual machines to process data. Charges depend on the type and number of virtual machines selected, as well as the hours they run.
- Databricks Units (DBUs): DBUs represent the processing capability on the Azure Databricks platform. Customers are billed based on the number of DBUs used. Different pricing tiers exist, which align with the performance level of the resources utilized.
- Storage Fees: Aside from computing costs, there are charges associated with how data is stored. Azure Storage options can influence the overall pricing, as certain types, like Azure Blob Storage, may incur different fees compared to Azure Data Lake Storage.
- Networking Costs: When data is transferred between Azure and other on-premises systems, additional costs might occur. It's essential to assess data transfer volumes to gauge potential expenses.
Understanding these components provides the foundation to manage budgets effectively, and can lead to cost-savings through informed decision-making. Each of these factors should be evaluated by businesses to tailor pricing decisions that match their unique analytics requirements.
Cost Breakdown by Resource Types
The cost breakdown according to resource types is essential for clarity when viewing pricing on Azure Databricks. Generally, this can be divided into categories:
- Virtual Machines: The core of the Azure Databricks functionality depends on virtual machines. For a data processing job, various types of VMs might be chosen—each with its own cost profile.
- DBU Consumption: Different workloads may lead to varying DBU consumption rates. For example:
- Data Storage: Allocating storage costs also varies greatly with choices made. Azure Data Lake typically incurs less overhead than Azure SQL Database when dealing with large volumes of unstructured data.
- Integration Features: Azure Databricks offers integrated services like Azure Machine Learning and Azure Synapse Analytics. While these integrations add functionality, they can also drive up costs depending on usage rates.
- For instance, Standard_DS3_v2 offers a good performance-to-cost balance for general analytics workloads.
- Consequently, opting for a high-performance VM may yield faster processing times but will also increase expenses significantly.
- Jobs optimized for job scheduling might incur lower DBU costs due to their predictable nature.
- Interactive Workloads, on the other hand, generally consume more DBUs since they require real-time processing.
A rigorous examination of these resource types helps applicants to strategize more effectively regarding what services they will most frequently utilize, ensuring that they are not overspending in areas that do not align with their business objectives.
Ultimately, comprehending both the components of pricing and the detailed cost breakdown enables businesses to make more informed decisions about how Azure Databricks aligns with their financial and operational goals.
Pricing Models Available
In the realm of cloud analytics, understanding the various pricing models is essential for businesses considering Azure Databricks. The choice of a pricing model impacts not only the immediate budget but also the long-term financial planning of an organization. Pricing models directly relate to how resources are consumed, with flexibility and predictability at the forefront of decision-making.
Azure Databricks offers primarily two types of pricing models: On-Demand Pricing and Reserved Capacity Pricing. Each model presents unique advantages and considerations suited for different business scenarios. Businesses need to evaluate their expected usage patterns, budget constraints, and overall resource requirements to select the most effective pricing model.
"Choosing the right pricing model can significantly alter the overall investment in cloud analytics, providing opportunities for cost savings."
On-Demand Pricing
On-Demand Pricing allows users to pay for the resources they consume as they go. This model suits businesses that have unpredictable workloads or are in the early stages of adopting data analytics, providing flexibility to scale services up or down based on real-time demand.
Benefits of On-Demand Pricing:
- Flexibility: Easily scales according to your project's needs.
- Cost-Effective for Variable Workloads: Pay only when you use the resources.
- No Long-Term Commitment: Ideal for short-term projects or trials.
However, while On-Demand Pricing offers accessible entry into Azure Databricks, it may lead to higher costs if workloads frequently spike. Hence, monitoring usage closely becomes a necessity to optimize spending.
Reserved Capacity Pricing
On the other hand, Reserved Capacity Pricing is an option for businesses that can predict their resource requirements over a more extended period. This model involves committing to a specific amount of capacity over a one-year or three-year term at a discounted rate.
Advantages of Reserved Capacity Pricing:
- Cost Savings: Generally, it offers a significant discount compared to On-Demand Pricing.
- Budget Planning: Facilitates better financial forecasting and stability.
- Guaranteed Resources: Ensures that resources are available when needed, which is important for high-demand periods.
That said, this commitment necessitates careful analysis of usage patterns. If usage falls below the reserved amount, businesses may end up overspending compared to the flexible options.
Factors Influencing Costs
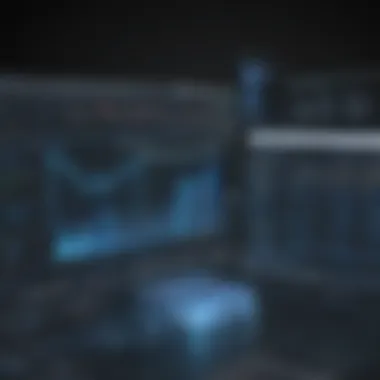
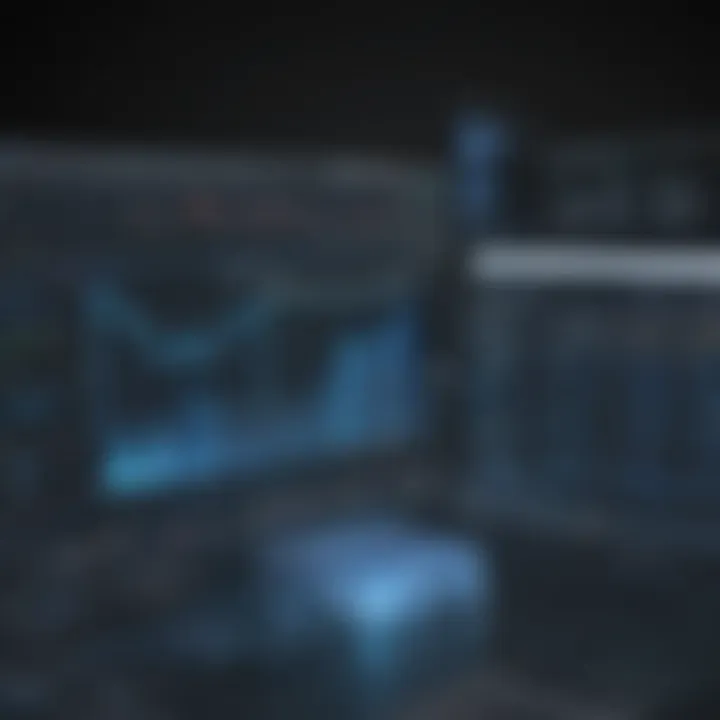
Understanding the factors influencing costs is crucial for businesses looking to utilize Azure Databricks. The pricing of this analytics platform can vary greatly based on several elements. These elements not only affect the overall expenses but also influence how effectively an organization can manage its data and analytics needs.
Two major components drive these costs: workload characteristics and data storage and transfer fees. Each of these components interacts with other factors, creating a complex pricing dynamics. By grasping these factors, businesses can better predict their expenses and budget accordingly, which is vital for small to medium-sized enterprises that often operate under stricter financial constraints.
Workload Characteristics
Workload characteristics play a significant role in determining the costs associated with Azure Databricks. It refers to the nature of the tasks that need to be executed within the platform. Different workloads have varying resource requirements, which can lead to differing costs.
- Data Volume: The amount of data being processed directly correlates with costs. Higher data volumes typically necessitate greater computational resources, leading to increased expenditures.
- Computational Complexity: More complex operations require more sophisticated resources. This includes machine learning algorithms, which may demand more CPU and memory, ultimately raising the costs.
- Concurrency Needs: The number of users and concurrent jobs also impacts pricing. Each additional user or parallel job consumes resources, and thus, users must consider this in their cost estimation.
These characteristics must be evaluated carefully. For instance, a simple batch processing task will typically incur lower costs than real-time analytics, which is inherently more resource-intensive. Understanding the specific workload can help organizations optimize their resource use, thus managing costs effectively.
Data Storage and Transfer Fees
Data storage and transfer fees are another fundamental aspect that influences the overall costs associated with Azure Databricks. This encompasses the cost of storing large datasets in Azure and transferring data in and out of the cloud platform.
- Storage Costs: The type of storage used will impact the pricing. Azure offers different storage solutions, with various pricing tiers. Choosing the most cost-effective option aligned with business needs is essential for budget management.
- Data Transfer Fees: Costs associated with data movement can accumulate quickly. For example, transferring significant amounts of data from Azure Databricks to external services may incur substantial fees. Understanding these fees is important for planning purposes.
- Types of Data: Different data types may have distinct storage requirements and costs. Businesses must consider whether their data is structured or unstructured, as this will influence the choice of storage solution.
Effectively managing data storage and transfer fees can be key differentiators in a company’s ability to maintain profitability while using Azure Databricks.
Combining insights from both workload characteristics and data storage can provide a much clearer picture of the expenses involved. Businesses are encouraged to analyze these factors systematically. The objective is to forecast potential costs accurately and to ensure that resources are used efficiently.
Cost Management Tips
Managing costs effectively is crucial for small to medium-sized businesses using Azure Databricks. Understanding how to monitor usage and optimize resource allocation can lead to significant savings. Careful consideration of cost factors can transform Azure Databricks from a merely beneficial platform to a financially sustainable choice for data analytics and processing.
Monitoring Usage and Costs
Monitoring usage and costs is an essential practice for any business leveraging Azure Databricks. Keeping track of who accesses the platform and how much resources they consume allows you to understand your spending patterns better. Azure provides various tools and reports, which can help you have a clear picture of your consumption and costs.
Some important methods for monitoring include:
- Azure Cost Management + Billing: This tool helps track expenditure trends specific to Azure Databricks and other Azure services.
- Databricks Cost Reports: These reports provide insights into usage aggregated across your clusters and jobs.
- Alerts and Notifications: Setting alerts can ensure that you are informed when your spending goes beyond your set budget.
Effective monitoring allows you to adjust or eliminate workloads that are too costly or unnecessary.
Optimizing Resource Utilization
Optimizing resource utilization is another vital aspect of managing costs on Azure Databricks. By ensuring that resources are utilized efficiently, businesses can avoid spending more than necessary. Here are some key strategies to consider:
- Cluster Sizing: Tailor the cluster size based on your workload requirement. Do not over-provision clusters, as that leads to unused capacity.
- Auto Termination of Clusters: Set up auto-termination to automatically shut down idle clusters. This prevents incurring costs when they are not in use.
- Spot Instances: Use spot instances for non-essential jobs to reduce costs significantly. This can lead to much lower pricing, albeit at the risk of instances being terminated.
Implementing these optimization strategies helps in achieving greater control over Azure Databricks costs.
"Proper cost management leads to smarter business decisions and allocation of resources."
Engaging in active monitoring and resource optimization can deliver immediate results in terms of cost efficiency. Combining these strategies yields not only better financial decisions but also a more sustainable use of cloud resources.
Comparing Azure Databricks Pricing with Competitors
The topic of comparing Azure Databricks pricing with its competitors is crucial for businesses seeking effective cloud analytics solutions. In today’s rapidly evolving tech landscape, organizations more than ever need to be strategic about where they allocate their resources. Understanding how Azure Databricks fares against its competitors in terms of pricing helps businesses make informed decisions that align with their financial goals and operational needs.
This section will explore specific elements of the pricing models, the advantages of various platforms, and considerations relevant to small and medium-sized enterprises as they navigate their options. Ultimately, a well-rounded comparison enhances an organization's ability to evaluate potential benefits while minimizing costs and risks involved in cloud analytics.
Key Competitors in the Market
When analyzing Azure Databricks, it’s important to acknowledge its main competitors. These platforms offer similar services and can help users better contextualize Azure's pricing structure. The following are notable alternatives:
- Amazon EMR: Amazon’s managed big data service, tailored for big data applications.
- Google BigQuery: A serverless data warehouse designed for real-time analytics and insights.
- Snowflake: A data cloud platform emphasizing scalability and ease of use with flexible pricing.
- Cloudera Data Platform: Integrates data engineering and machine learning frameworks for hybrid cloud environments.
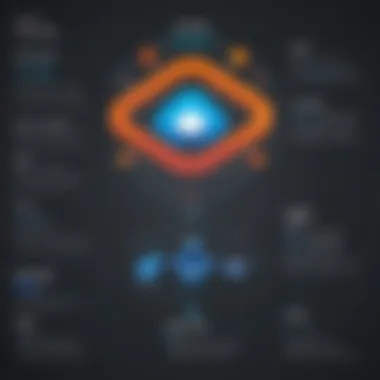
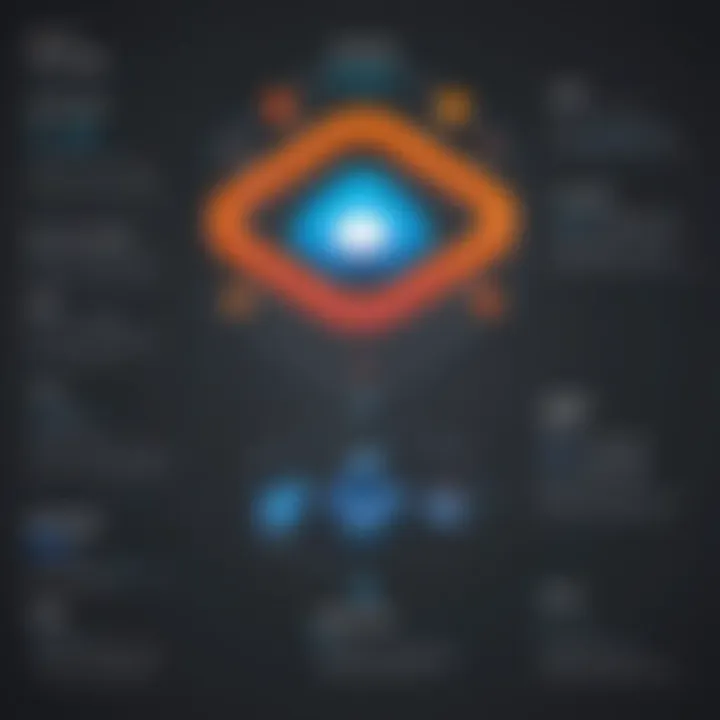
Each of these platforms has its own pricing structure, strengths, and weaknesses. For example, Amazon EMR can be more cost-effective for large-scale data processing, while Google BigQuery charges based on query size, which may be better for businesses with fluctuating workloads.
Cost Comparison Analysis
Comparative analysis of costs between Azure Databricks and its competitors is essential. Here are a few factors to consider when conducting this analysis for effective decision-making:
- Pricing Models: Each service provides different models. Azure Databricks offers on-demand pricing as well as reserved capacity pricing. Amazon EMR typically charges by the hour for each instance.
- Usage Patterns: Costs can significantly vary depending on workload types, such as batch versus streaming analytics. Understanding workload patterns is vital in evaluating total expenses.
- Scalability and Flexibility: Some platforms may offer better scalability options, impacting costs as needs change over time.
- Hidden Costs: Features such as data ingress and egress charges can add hidden costs in competitor pricing structures.
By compiling and analyzing these details, organizations can formulate a cost-benefit analysis that aligns with their specific needs.
"In evaluating cloud solutions, it is not only about direct costs but also considering the total cost of ownership over time."
This thoughtful approach helps ensure that organizations not only select a competitive pricing model but also integrate a comprehensive solution that satisfies their long-term analytical demands.
Real-World Use Cases
Understanding the real-world applications of Azure Databricks is essential for businesses considering this powerful analytics platform. Industry leaders are turning to Azure Databricks to address varying demands across sectors like finance, healthcare, and retail. Each sector leverages the platform differently, showcasing its versatility and the scalability it offers.
Industry Applications of Azure Databricks
Azure Databricks is utilized in diverse industries.
- Financial Services: In finance, institutions analyze massive datasets to detect fraud, manage risk, and enhance customer experience. Azure Databricks enables real-time data processing, ensuring that financial firms can react quickly to market changes.
- Healthcare: For healthcare providers, handling patient data securely and efficiently is paramount. Azure Databricks supports machine learning models that can predict patient outcomes, optimize treatment plans, and manage administrative tasks more effectively than traditional methods.
- Retail: Retailers apply Azure Databricks to gain insights into customer preferences and optimize inventory management. Analyzing sales trends and customer feedback allows businesses to make informed decisions, enhancing their competitiveness in the marketplace.
Cost Implications in Industry Scenarios
When adopting Azure Databricks, businesses must consider the costs associated with its real-world applications. The financial investment varies depending on use cases:
- Initial Setup Costs: Setting up Azure Databricks can involve substantial initial expenses, particularly if significant data migration is necessary. Companies need to weigh these costs against the expected improvements in operational efficiency.
- Ongoing Operational Costs: The ongoing charges depend on the services used and the volume of data processed. For instance, companies might experience higher costs when using advanced analytics or machine learning features, which require more resources.
- Cost Savings through Efficiency: Despite these costs, many organizations realize that Azure Databricks can lead to long-term savings. The increased speed of data processing and improved decision-making capabilities can offset initial expenses, making it a worthwhile investment.
"Investing in Azure Databricks often leads to enhanced operational efficiency, which can outweigh initial setup and ongoing costs."
Navigating these real-world use cases and their associated costs is crucial for businesses. They enable organizations to assess the value Azure Databricks can deliver within their specific context. Businesses must analyze costs carefully to ensure they align with the anticipated benefits, positioning themselves for success.
Frequently Asked Questions
In the context of Azure Databricks pricing, Frequently Asked Questions (FAQs) provide clarity for businesses and individuals exploring this powerful analytics platform. Understanding commonly raised queries can significantly ease the decision-making process. It is essential for potential users to grasp the nuances of pricing structures and billing cycles to avoid unexpected expenses. FAQs create an accessible entry point for users to gather necessary insights quickly. They can address misconceptions and deliver clarity about financial commitments related to the service.
Common Queries on Pricing
Potential users often have several questions regarding Azure Databricks pricing. Here are some commonly asked questions:
- What factors impact the pricing of Azure Databricks? Prices can vary based on several factors, including the selected instance type, the number of compute nodes, and the storage used.
- Is there a trial period available? Azure Databricks typically offers a trial period. This can be valuable for businesses to assess the platform's capabilities without incurring costs.
- How can I predict costs accurately? Cost prediction involves examining expected workloads, the type of data processed, and potential scaling needs that might arise during peak usage.
- Are there any hidden costs? It is crucial to read the fine print regarding additional fees for data transfer or excessive usage that exceeds the initially budgeted amount.
These queries signal larger concerns that need addressing when considering Azure Databricks. They encompass the essential components of pricing that, when understood, empower businesses to forge ahead with confidence.
Understanding Billing Cycles
Billing cycles with Azure Databricks are a critical part of managing overall expenses. Knowing how and when charges are applied can inform budgeting practices effectively.
Typically, Azure Databricks follows a monthly billing cycle. Users are charged based on their resource consumption during that period. Here are key points about billing cycles that users should note:
- Monthly Billing: Charges are usually calculated at the end of each month. This aligns with most cloud services, making it easier for financing and budgeting.
- Usage Reports: Azure provides detailed usage reports that help customers track their expenditures. These reports can offer insights into deployed resources and their corresponding costs.
- Notifications: Users can set up alerts for spending milestones to help manage their budget effectively. This prevents overruns and ensures businesses stay on track financially.
In summary, understanding billing cycles is just as imperative as grasping the general pricing structure. This knowledge allows businesses to approach Azure Databricks with a greater degree of financial awareness.
Closure
The conclusion of an article about Azure Databricks pricing is a vital section. It encapsulates the essential points discussed, crafting a clear summary for the readers. This section not only reaffirms the key takeaways but also emphasizes the relevance of the pricing structures presented. By summarizing the complexities of Azure Databricks pricing, readers can align their understanding with practical applications.
When we look at Azure Databricks pricing, we acknowledge multifaceted components such as resource allocation, usage patterns, and storage considerations. These factors have immense implications for any business, particularly for small to medium-sized enterprises, where every dollar spent on cloud solutions needs justification.
One benefit of understanding this pricing model is enhanced budgeting. Companies can better anticipate costs through careful planning, which helps in optimizing expenditures. Recognizing how various factors like workload characteristics and data transfer fees influence overall costs can lead to informed decisions. This clarity can guide businesses in determining the most suitable pricing model that aligns with their operational needs.