Understanding Iterable AI: Revolutionizing Business Efficiency
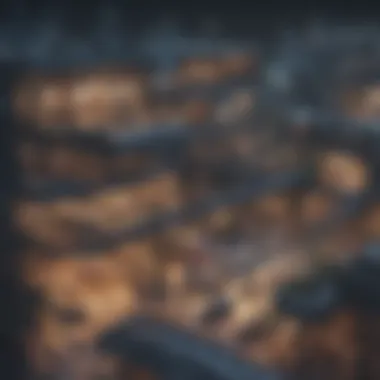
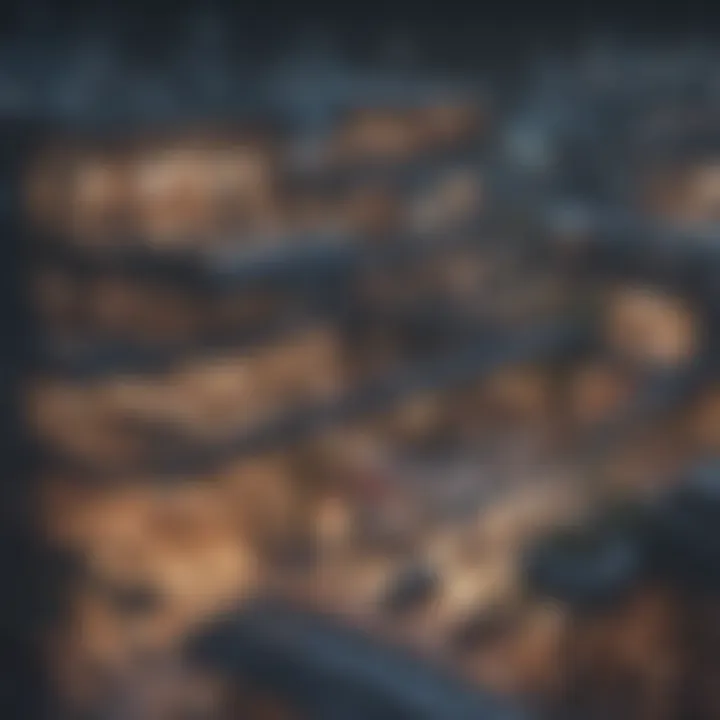
Intro
As businesses strive to remain competitive in an ever-evolving landscape, the adoption of advanced technologies like Iterable AI has emerged as a significant trend. Iterable AI integrates artificial intelligence capabilities with operational processes to enhance efficiency and productivity. This article explores the intricate nature of Iterable AI, its core functionalities, and its implications for modern businesses.
Iterable AI is fundamentally reshaping how enterprises operate. By automating tasks and providing data-driven insights, it aids in decision-making, marketing strategies, and customer interaction. The following sections will dissect its core features, significant advantages, and potential drawbacks.
Key Features of the Software
Overview of Core Functionalities
Iterable AI is equipped with several essential functionalities that empower businesses to optimize their operations. These include data processing capabilities, predictive analytics, and personalized customer engagement tools.
- Data Processing: This functionality allows businesses to efficiently analyze vast data sets, transforming raw data into actionable insights.
- Predictive Analytics: Leveraging machine learning algorithms, Iterable AI predicts future trends based on historical data, aiding in informed decision-making.
- Personalized Engagement: The software assists companies in tailoring their marketing efforts by segmenting audiences and delivering customized communications.
Unique Selling Points
Iterable AI offers unique advantages that distinguish it from other solutions in the market. These include:
- User-Friendly Interface: Easy for non-technical users, enhancing adoption across various business roles.
- Seamless Integration: Works well with existing software systems, minimizing disruption during implementation.
- Scalability: Capable of growing with the business, ensuring continued effectiveness as needs evolve.
In-Depth Software Analysis
Pros and Cons
Analyzing the strengths and weaknesses of Iterable AI is crucial for understanding its suitability for specific business needs.
Pros:
- Increased Efficiency: Automates repetitive tasks, freeing staff for strategic tasks.
- Data-Driven Decisions: Encourages reliance on analytics over intuition, improving outcomes.
Cons:
- Cost: Initial investments can be high, particularly for small businesses.
- Complexity: Might require training to maximize use of advanced features.
Performance Metrics
When evaluating the performance of Iterable AI, consider several key metrics:
- Return on Investment (ROI): Measured through improved sales and reduced operational costs.
- User Engagement Rates: Increases in customer interaction can indicate the effectiveness of personalized campaigns.
- Data Accuracy: The reliability of insights drawn from data is essential.
"The best technology will adapt to the needs of the user, ensuring a seamless experience that prioritizes efficiency."
In summary, Iterable AI presents many benefits designed to enhance operational capabilities. As businesses navigate the complexities of modern markets, understanding how to leverage these tools is essential for success.
Intro to Iterable AI
In our rapidly evolving technological landscape, understanding Iterable AI is crucial for businesses aiming to maintain a competitive edge. This concept encapsulates a range of applications and methodologies that enhance efficiency and productivity across various sectors. Businesses today are exploring ways to integrate Iterable AI into their operations to improve decision-making, automate processes, and deliver better customer experiences. The exploration of Iterable AI not only sheds light on its practical benefits but also provides insights into the historical context and current trends shaping its adoption.
Defining Iterable AI
Iterable AI refers to the methodologies and frameworks that allow artificial intelligence systems to learn from data in an iterative fashion. This iterative learning process enables machines to refine their algorithms based on feedback and new data inputs. It emphasizes continuous improvement, essential for businesses looking to adapt quickly to changes in market conditions and consumer behavior.
At its core, Iterable AI leverages algorithms that analyze vast datasets, offering insights that drive informed decision-making. These insights are pivotal for small to medium-sized businesses and entrepreneurs, as they enable tailored solutions that fit specific operational needs.
It is worth noting that
- Iterable AI can be applied in various contexts, from marketing to inventory management.
- Its iterative nature ensures that models evolve over time, becoming increasingly effective at performing their designated tasks.
Historical Context and Development
The development of Iterable AI stems from advances in machine learning and data analytics, dating back several decades. Initially, machine learning was largely focused on static models. Today, the emphasis has shifted toward adaptive systems that learn continuously.
The integration of big data has played a significant role in this evolution. As more data became available, businesses began to recognize its potential for informing strategies and optimizing operations.
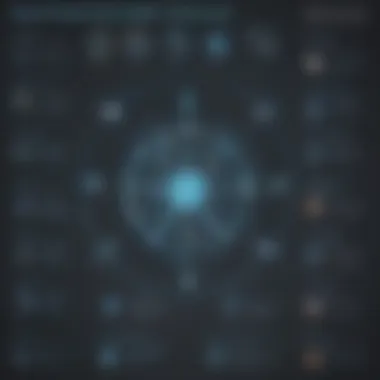
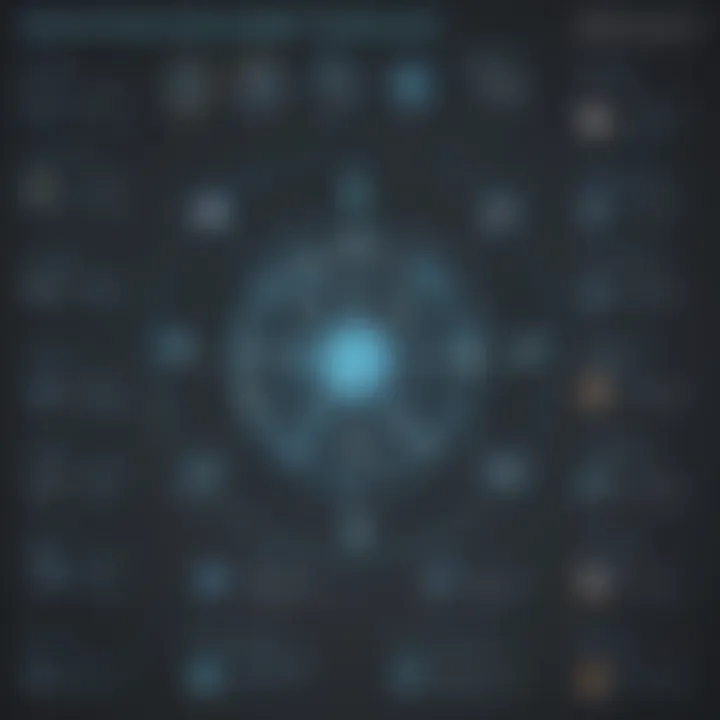
Several key developments contribute to the rise of Iterable AI:
- Increased computing power: This has made it feasible to process and analyze large datasets efficiently.
- Advancements in algorithms: The introduction of more robust algorithms enables faster and more accurate learning.
- Widespread data availability: Sources such as social media, IoT devices, and transaction data have enriched the datasets available for analysis.
This historical progression highlights not only how Iterable AI emerged but also its pivotal role in shaping modern business strategies. Understanding this foundation is critical for decision-makers looking to leverage Iterable AI effectively in their operations.
"The past serves as a roadmap for the future, guiding companies in their journey toward AI integration."
Core Principles of Iterable AI
Understanding the core principles of Iterable AI is essential for businesses aiming to harness its full potential. These principles underscore the framework that allows Iterable AI to function effectively. They encapsulate the processes that bolster efficiency, drive performance, and refine the overall user experience.
Data Processing and Management
Data serves as the foundation upon which Iterable AI operates. Proper data processing and management ensures that the information collected is cleansed, structured, and readily accessible. This encompasses several specific elements:
- Data Collection: Gathering data from various sources, such as customer interactions, sales transactions, and market trends.
- Data Quality Assurance: Implementation of techniques to cleanse data, addressing inaccuracies or duplicates to maintain integrity.
- Storage Solutions: Utilizing various systems for secure storage, allowing easy retrieval and analysis. Storage can be cloud-based like AWS or Azure, which scales efficiently with business needs.
By formalizing these elements, businesses can improve decision-making processes. Quality data management positively impacts other functions as well, including analytics, marketing strategies, and customer service, tying back into the core principles of Iterate AI.
Algorithm Efficiency
Algorithms are central to the functionality of Iterable AI. The effectiveness of these algorithms in processing data and producing actionable insights directly relates to overall efficiency. Considerations for algorithm efficiency include:
- Performance Metrics: Assessing how well algorithms perform against established benchmarks.
- Adaptability: Algorithms must be able to adjust based on changing inputs or conditions, ensuring relevance and accuracy.
- Resource Allocation: Efficient algorithms minimize the use of computational resources, reducing operating costs for businesses.
Efficient algorithms mean more timely insights, which can lead to quicker responses in strategic business decisions. Optimization of these algorithms is vital, especially for small and medium-sized businesses where resources may be limited.
User Interaction Models
User interaction models play a crucial role in the application of Iterable AI. They determine how end-users will engage with AI systems. Important elements to consider include:
- User Experience Design: Focusing on a clear and intuitive interface enhances user satisfaction and encourages adoption.
- Personalization: AI systems should adapt to user preferences, enhancing the relevance of interactions. This fosters deeper connections with customers.
- Feedback Mechanisms: Providing users with ways to give feedback aids in refining the AI's responses and functionalities.
Engaging with users effectively not only boosts productivity but also builds lasting relationships, reducing customer churn.
"The success of Iterable AI hinges on its ability to process data, run efficient algorithms, and foster meaningful interactions with users."
Overall, these core principles create a robust framework for Iterable AI, offering businesses the tools necessary to thrive in increasingly competitive landscapes.
Applications of Iterable AI
The applications of Iterable AI are vast and critical for modern businesses. They represent a tangible way that this technology can improve operational efficiency and decision-making processes. As companies increasingly rely on data-driven strategies, the incorporation of Iterable AI in various sectors becomes essential. Organizations can better understand their customer base, optimize their resources, and enhance their overall performance through targeted applications.
Marketing Automation
Marketing automation is a primary application of Iterable AI. It allows businesses to streamline their marketing efforts, saving time and resources while increasing engagement with customers. AI can analyze customer behavior to segment audiences effectively. This segmentation leads to personalized marketing strategies, resulting in higher conversion rates. Tools such as HubSpot and Marketo utilize Iterable AI for creating automated email campaigns that adapt based on consumer interaction.
Another advantage is the ability to measure the effectiveness of marketing campaigns in real time. Companies can adjust their strategies based on analytics, ensuring that marketing budgets are efficiently allocated. This flexibility and responsiveness can result in significant competitive advantages in todayβs fast-paced market.
Customer Relationship Management
In the realm of customer relationship management (CRM), Iterable AI plays an integral role. Businesses can harness AI to better understand customer preferences and behaviors. By utilizing platforms like Salesforce, organizations can collect relevant data that help in forecasting future customer interactions.
These insights allow companies to personalize communication strategies, fostering deeper relationships with their clients. Furthermore, AI-enhanced CRM can predict potential issues before they escalate, enabling proactive support and retention efforts. Providing personalized customer service has become a cornerstone in maintaining loyalty and satisfaction.
Inventory Management Solutions
Effective inventory management can be achieved through the integration of Iterable AI. Businesses, especially in retail and e-commerce, face challenges in predicting demand, leading to either surplus stock or shortages. AI solutions can analyze historical data and market trends, helping organizations optimize their inventory levels.
By tracking stock movement in real time, systems like Oracle NetSuite can alert businesses when certain products are running low or need reordering. This responsiveness minimizes waste and ensures that businesses can meet customer demands promptly. Thus, initial costs of implementing such innovations can be outweighed by the savings derived from reduced overstock and lost sales.
Predictive Analytics in Business
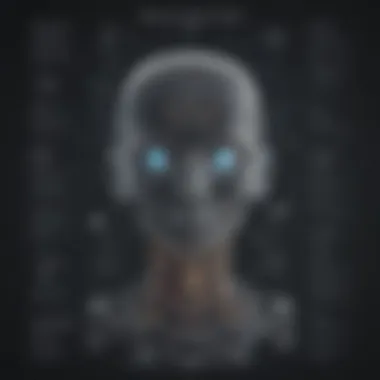
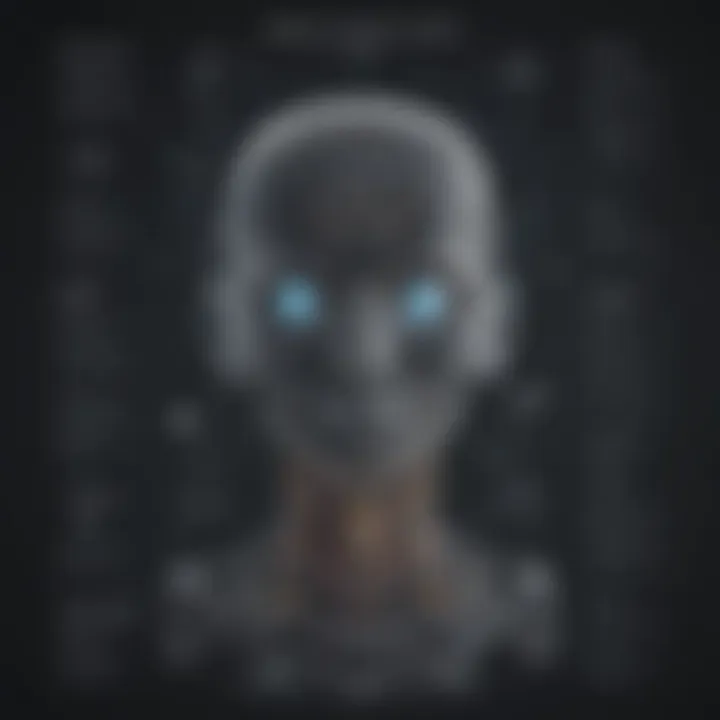
Predictive analytics is a significant application of Iterable AI. It allows businesses to foresee trends and make informed decisions based on data forecasts. This capability is especially crucial in industries that are subject to rapid change, such as finance or technology.
Tools like Microsoft Azure and IBM Watson enable organizations to utilize predictive models to analyze vast datasets. These insights inform strategic planning, whether it's entering new markets or investing in product development. By leveraging predictive analytics, businesses can remain ahead of their competitors, adapting swiftly to changes in consumer behavior.
"In the evolving landscape of business, harnessing the power of Iterable AI for predictive analytics is no longer a luxury but a necessity for survival."
Technical Architecture of Iterable AI
The technical architecture of Iterable AI is pivotal in understanding how businesses can leverage this technology effectively. It encompasses the software systems, frameworks, and methodologies that allow Iterable AI to function optimally. In an age where data drives decision-making, having a solid architectural foundation ensures that businesses can harness the full potential of Iterable AI tools.
Machine Learning Frameworks
In the realm of Iterable AI, machine learning frameworks serve as the backbone for processing data and executing algorithms. These frameworks dictate how data is handled, from training models to deploying them for real-world applications.
Some of the most notable frameworks include TensorFlow, PyTorch, and Scikit-learn. Each framework possesses unique attributes that cater to different business needs. For instance, TensorFlow offers scalability and is suited for complex projects, while Scikit-learn is user-friendly and ideal for beginners or smaller scale applications.
The choice of framework impacts not only the performance but also the ease of integration with existing systems. The efficiency of the machine learning framework determines the overall accuracy and speed of the Iterable AI solutions deployed. Selecting the appropriate framework is crucial for businesses to ensure they receive the best results.
Integration with Existing Systems
Integration is a critical consideration when deploying Iterable AI solutions. Businesses often operate with legacy systems that handle various processes. Thus, ensuring seamless interoperability between these systems and the new AI frameworks is essential for operational efficiency. This can include everything from customer databases to financial reporting tools.
The integration phase typically involves the use of APIs and middleware, which facilitates communication between disparate systems. Businesses should also consider the data format compatibility to ensure successful data transfers. A robust integration strategy minimizes disruption and allows for a smoother transition to AI-driven solutions.
Data Sources and Management
The success of Iterable AI hinges significantly on the quality and management of data sources. Data feeds can be highly diverse, including structured data from databases, unstructured data from social media, or semi-structured data like emails.
Effective data management practices ensure that data is accurate, timely, and relevant. Implementing a clear data governance strategy is crucial. This involves setting standards for data collection, storage, and utilization.
Moreover, utilizing tools for data cleaning and transformation, such as Apache Spark or Azure Data Factory, can significantly enhance the data preparation process. Well-managed data sources improve the performance of Iterable AI systems, leading to more accurate predictions and insights.
"The architecture of Iterable AI revolves around data and how effectively it can be managed and utilized in real-time scenarios."
Impact of Iterable AI on Business Efficiency
The integration of Iterable AI into business operations signifies a fundamental shift in how organizations approach efficiency. This AI technology is not merely a tool; it transforms process optimization and decision-making by harnessing vast amounts of data. Implementing Iterable AI can streamline workflows, leading to increased productivity and a heightened competitive edge. For small to medium-sized businesses, embracing this technology means not just staying relevant but also maximizing their operational capabilities.
Cost Reduction Strategies
Implementing Iterable AI often leads to significant cost-saving opportunities. For instance, automating routine tasks allows businesses to redirect their human resources to more strategic initiatives. By minimizing manual interventions, companies reduce the likelihood of errors, which can be costly. Companies can also benefit from improved inventory management, where AI algorithms predict demand and optimize stock levels, thereby reducing holding costs.
- Automation of repetitive tasks: This process cuts down on labor costs and reduces time spent on low-value activities.
- Supply chain optimization: Predictive algorithms can enhance ordering processes, preventing overstock and stockouts.
- Resource allocation: AI helps in efficiently allocating resources, ensuring that funds and personnel are utilized effectively.
According to reports, businesses that utilize AI for cost management strategies report a decrease in operational expenses while enhancing productivity.
Improved Decision-Making Processes
The analytical capabilities of Iterable AI provide a substantial advantage for decision-making within organizations. With data-driven insights, businesses can make decisions more swiftly and with greater precision. Iterable AI aggregates data from multiple sources, analyzing trends and patterns that might not be visible through traditional methods. This informed approach helps decision-makers to not only react to current situations but also to forecast future trends.
- Data interpretation: AI systems can process complex datasets to provide clear, actionable insights.
- Scenario analysis: Businesses can run different scenarios and assess outcomes, enabling better strategic planning.
- Real-time feedback: Continuous analysis allows for timely interventions, reducing risks associated with poor decision-making.
The ability to make decisions backed by comprehensive data analysis significantly enhances organizational agility.
Enhancing Customer Experiences
Iterable AI has a profound impact on customer experience, which is increasingly becoming a crucial differentiator in competitive markets. By leveraging AI capabilities, businesses can personalize offerings and improve engagement with customers. Understanding customer behavior through data analysis allows organizations to tailor their services and communications effectively.
- Personalized marketing: AI enables segmentation and targeted marketing efforts, ensuring that messages reach the right audiences.
- Customer support automation: Chatbots and virtual assistants powered by AI provide instant support, improving response rates and customer satisfaction.
- Feedback mechanisms: AI analyzes customer feedback efficiently, helping businesses to adjust their strategies to meet client needs better.
"Companies that prioritize customer experience through AI not only enhance loyalty but also drive revenue growth over time."
Challenges and Limitations of Iterable AI
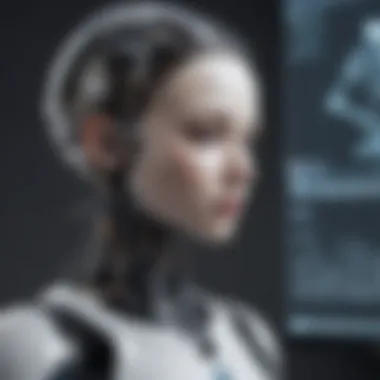
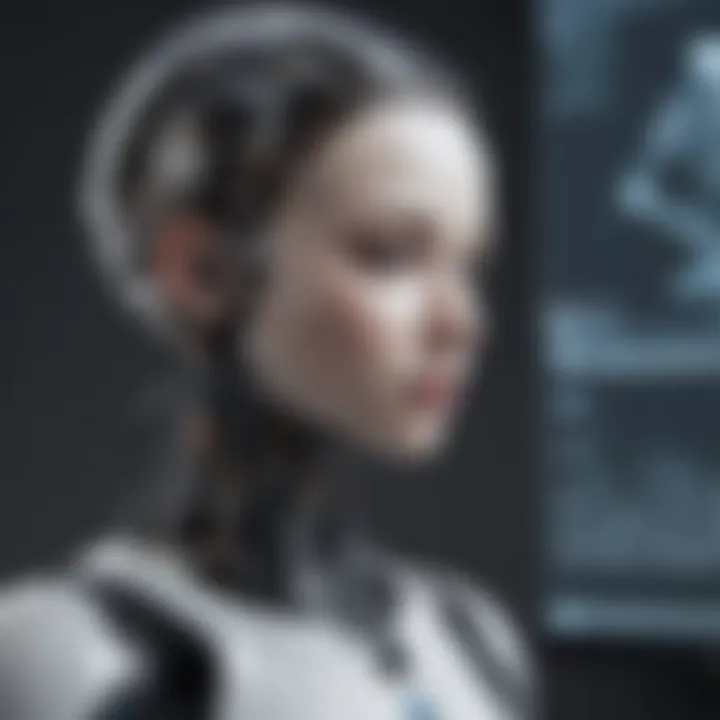
Utilizing Iterable AI, while promising, comes with a set of inherent challenges and limitations. Understanding these aspects is critical for businesses considering its implementation. By recognizing potential pitfalls, companies can prepare better, mitigate risks, and ensure a smoother integration into their operational frameworks. Addressing these limitations fosters a more sustainable approach to leveraging Iterable AI for enhanced efficiency and decision-making.
Ethical Implications of Iterable AI
The integration of Iterable AI in business operations raises significant ethical considerations. These implications are essential to understand as they can influence not only the decision-making processes but also the public perception of the businesses utilizing such technologies. Addressing these ethical concerns ensures that businesses operate within a framework that prioritizes fairness and accountability.
Bias in Algorithms
One of the most pressing issues in AI technology is the potential for bias within algorithms. Bias can emerge from various sources including the data used for training models. If the training data reflects historical prejudices or is not representative of the entire population, the AI systems may produce outcomes that inadvertently favor one group over another.
It is important for businesses to regularly review and audit their algorithms to identify sources of bias. This process includes understanding the demographic data involved and the implications of biased results. Companies should also implement diverse hiring practices, ensuring the teams that build these algorithms contain a range of perspectives, which can contribute to more equitable technology.
Transparency and Governance
Transparency in AI systems is critical for fostering trust among users and stakeholders. Businesses need to provide clear explanations of how their AI systems operate and make decisions. This includes documenting the sources of their data, the mechanisms of the algorithms, and the criteria for decision-making.
Governance structures must be put in place to oversee the use of Iterable AI. Clear guidelines and accountability measures should be established to address any misuse of AI technology. Regular evaluations and an open dialogue with stakeholders can contribute to a governance framework that places ethical considerations at its core. This will not only help manage risks but also enhance the overall credibility of AI applications within organizations.
"Ensuring ethical use of AI transforms potential risks into opportunities for responsible innovation."
In summary, understanding the ethical implications of Iterable AI is imperative for businesses aiming to adopt these technologies effectively. By addressing bias and emphasizing transparency, organizations can not only enhance their operational efficiency but also preserve their reputation in a rapidly evolving technological landscape.
Future Trends of Iterable AI
The landscape of Iterable AI continues to evolve rapidly, and its future trends play a pivotal role in shaping its application in business processes. Understanding these developments is crucial for organizations aiming to enhance their operational efficiency and stay competitive in their respective markets. By focusing on emerging technologies and market predictions, businesses can strategize effectively and embrace new opportunities.
Emerging Technologies and Innovations
Advancements in technology are driving the evolution of Iterable AI. Some of these emerging technologies include:
- Natural Language Processing (NLP): This enables machines to understand and generate human language. Better NLP can improve customer interaction and support systems.
- Deep Learning: A subset of machine learning that mimics human brain functionality, allowing for more accurate predictions and insights from data.
- Internet of Things (IoT): Integration of IoT devices with Iterable AI leads to more efficient data collection and analysis, giving businesses real-time insights.
These technologies present distinct benefits:
- Increased accuracy in data interpretation reduces human error.
- Automation of repetitive tasks enhances productivity.
As these innovations permeate various sectors, businesses that adopt them early can capitalize on a competitive edge, optimizing their resources and processes effectively.
Market Predictions and Growth
The market for Iterable AI is expected to expand significantly in the coming years. Analysts predict a compound annual growth rate (CAGR) of over 30% in the next five years. Several factors contribute to this growth:
- Rising Demand for Automation: Companies increasingly seek automated solutions to streamline business operations, leading to higher adoption rates.
- Increased Investment in AI Technologies: More investments from both public and private sectors are propelling developments in AI, which, in turn, fosters a more robust ecosystem for Iterable AI applications.
- Focus on Customization: Businesses are moving towards more personalized experiences for their customers. Iterable AI can analyze customer behavior and preferences, allowing for tailored marketing and sales approaches.
"As organizations recognize the impact of Iterable AI on their bottom lines, the pace of investment is expected to rise significantly."
By keeping an eye on these trends, companies can align their strategies accordingly, ensuring they remain relevant in an increasingly competitive market. Embracing future trends in Iterable AI is not just an opportunity but a necessity for sustainable growth.
Finale
In the landscape of modern business, the integration of Iterable AI represents not only an innovation but a paradigm shift. This article has explored various dimensions of this technology, encapsulating its capabilities and significance in enhancing operational efficiency.
Summarizing Key Insights
Throughout the discussion, several pivotal points emerged about Iterable AI:
- Efficiency Gains: Iterable AI presents a unique ability to streamline processes. By automating routine tasks, it enables businesses to allocate resources more effectively and focus on high-value activities.
- Diverse Applications: The versatility of Iterable AI allows it to touch various facets of a business, including marketing automation and predictive analytics. This breadth of application is essential for adapting to dynamic market demands.
- Ethical Considerations: Issues like algorithmic bias and the need for transparency in AI operations require careful attention. Businesses must build frameworks to address these challenges while leveraging technology.
- Future Trends: Understanding the trajectory of Iterable AI is crucial. Emerging technologies will continue to transform businesses, and staying ahead of these changes can provide a competitive edge.
The insights derived showcase not only the benefits but also the considerations necessary for successful implementation.
Final Thoughts on Implementing Iterable AI
Implementing Iterable AI requires a careful approach. Businesses, especially small and medium-sized ones, must evaluate their specific needs and capabilities. A successful deployment can lead to remarkable improvements in productivity and decision-making.
- Strategic Planning: Identifying clear goals for using Iterable AI will allow businesses to tailor their strategies effectively. This clarity will facilitate smoother integration with existing systems.
- Training and Development: The skill gaps in the workforce need address. Investing in training is vital to ensure personnel can fully utilize the advantages of Iterable AI tools.
- Measuring Success: Continuous assessment of the AI's impact on business operations is critical. Metrics should be established to track progress and guide future iterations of the technology.
"The future thrives on data-driven decisions amplified by technologies like Iterable AI. Adopt wisely, and the rewards can be substantial."
In summary, the potential of Iterable AI is vast, and when applied thoughtfully, it can significantly elevate a company's operational efficiency and market competitiveness. Businesses are encouraged to embrace this technology and adapt to the unfolding changes in the digital landscape.